Goldman Sachs Deciphers Trump's Preferred Oil Price Through Social Media Analysis
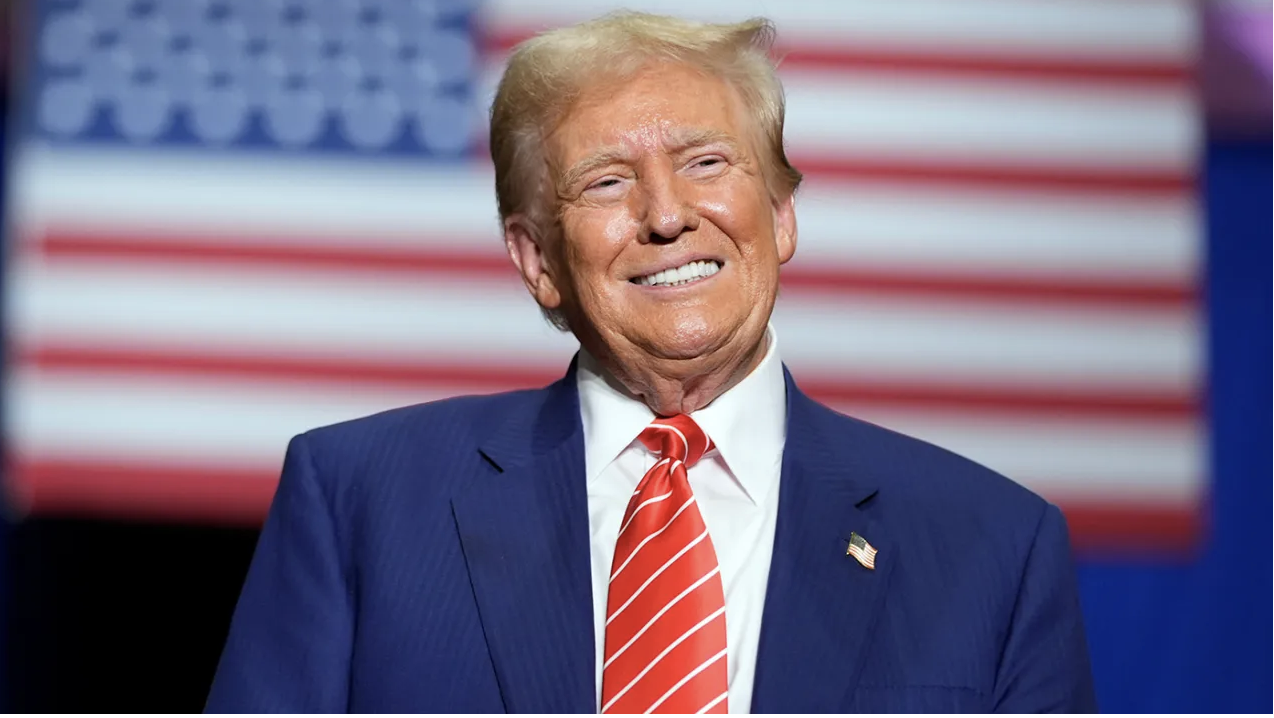
Table of Contents
The Methodology Behind Goldman Sachs's Social Media Analysis
Goldman Sachs's endeavor to gauge President Trump's preferred oil price involved a multi-faceted approach to social media analysis. This involved meticulous data collection, advanced sentiment analysis techniques, and rigorous correlation analysis.
Data Collection and Sources
The analysis likely encompassed a vast quantity of data sourced from various social media platforms.
- Specific social media platforms used: Twitter, Facebook, potentially including less prominent platforms like Gab or Parler, given Trump's usage patterns.
- Timeframe of data collection: The data collection likely spanned Trump's presidency (2017-2021), possibly extending to cover periods before and after his time in office to establish baselines and assess evolving sentiment.
- Data cleaning processes: This crucial step involved removing irrelevant data, dealing with duplicates, and handling inconsistencies to ensure data accuracy and reliability. This likely included filtering out bots and spam accounts.
- Challenges in verifying data authenticity: Identifying and filtering out misinformation and fake news was a major hurdle, impacting the accuracy of sentiment analysis. Verifying the authenticity of accounts and posts presented a significant challenge.
Sentiment Analysis Techniques
Determining the sentiment expressed towards oil prices within the context of Trump's statements required advanced techniques.
- Natural language processing (NLP): Sophisticated NLP algorithms were used to process the vast amount of text data, identifying keywords and phrases related to oil prices and Trump's commentary.
- Machine learning algorithms used: Machine learning models were likely employed to classify the sentiment expressed as positive, negative, or neutral. These models would learn from labelled data to improve their accuracy over time.
- Specific metrics used to measure sentiment (e.g., polarity scores): Metrics like polarity scores (measuring the degree of positivity or negativity) and subjectivity scores (measuring the degree of opinion versus factual statement) were used to quantify the sentiment.
Correlation Analysis
Goldman Sachs likely employed statistical methods to correlate the sentiment analysis results with actual oil price movements.
- Statistical methods employed: Regression analysis, time series analysis, and potentially other econometric techniques were likely employed to assess the relationship between sentiment and oil price changes.
- Identification of significant correlations: The analysis aimed to identify statistically significant correlations between positive sentiment towards higher oil prices (or negative sentiment towards lower prices) and subsequent price increases.
- Challenges in establishing causality: It's crucial to note that correlation doesn't equal causation. Other factors like global supply and demand, geopolitical events, and economic indicators also significantly influence oil prices.
Goldman Sachs's Findings: Uncovering Trump's Ideal Oil Price Range
While Goldman Sachs hasn't publicly released their specific methodology or findings, speculation suggests the analysis uncovered a preferred oil price range.
The Identified Price Range
The purported findings suggest a specific range, though exact figures remain unconfirmed.
- Specific price range: Speculation points towards a range that benefited American energy producers, possibly between $60 and $80 per barrel (USD). This range is purely hypothetical based on external speculation, not confirmed Goldman Sachs data.
- Justification for the range: The reasoning would be based on interpreting Trump's statements and tweets expressing support for energy independence and American oil production. Higher prices would generally favor domestic producers.
- Supporting evidence from social media analysis: The evidence for this conclusion would rest on a correlation between positive social media sentiment towards higher oil prices (associated with Trump's statements) and observed price movements.
Implications for the Energy Market
The implications of such a finding extend across the energy market landscape.
- Impact on oil production: A higher preferred oil price would incentivize increased domestic oil production in the United States.
- Effect on consumer prices: Higher oil prices generally lead to increased gasoline and energy costs for consumers.
- Influence on international relations: Trump's preferred oil price could have influenced US foreign policy, impacting relations with OPEC nations and other energy-producing countries.
- Potential market volatility: The influence of political sentiment on oil prices can introduce significant market volatility and unpredictability.
Criticisms and Limitations of the Social Media Analysis
Despite its innovative approach, Goldman Sachs's analysis isn't without limitations.
Bias and Subjectivity
The analysis is susceptible to various biases.
- Potential for algorithmic bias: The algorithms used in sentiment analysis can inherit biases present in the training data, potentially leading to skewed results.
- Challenges in accurately interpreting nuanced language: Sarcasm, irony, and other linguistic subtleties can be difficult for algorithms to interpret accurately.
- Influence of fake news or bots: The presence of fake news, bots, and coordinated disinformation campaigns can significantly distort the sentiment expressed on social media.
Causality vs. Correlation
It's crucial to reiterate the importance of distinguishing correlation from causation.
- Other factors influencing oil prices: Numerous factors, including global supply and demand, geopolitical instability, and economic conditions, significantly affect oil prices.
- Limitations of correlation analysis: Correlation analysis can only demonstrate a statistical association, not a causal relationship.
- Need for further research to confirm causality: Further research utilizing more robust methodologies is needed to establish a clear causal link between Trump's social media activity and oil price movements.
Conclusion
Goldman Sachs's purported use of social media analysis to uncover former President Trump's ideal oil price represents a novel approach to analyzing the influence of political sentiment on the energy market. While the analysis offers intriguing insights, it's crucial to acknowledge the limitations inherent in relying on social media data and sentiment analysis algorithms. The potential for bias, the difficulty in establishing causality, and the impact of misinformation necessitate careful interpretation of the findings. Understanding how social media analysis, like that conducted by Goldman Sachs, can be used to interpret political influence on the oil price is crucial. Stay tuned for future insights into this evolving field.
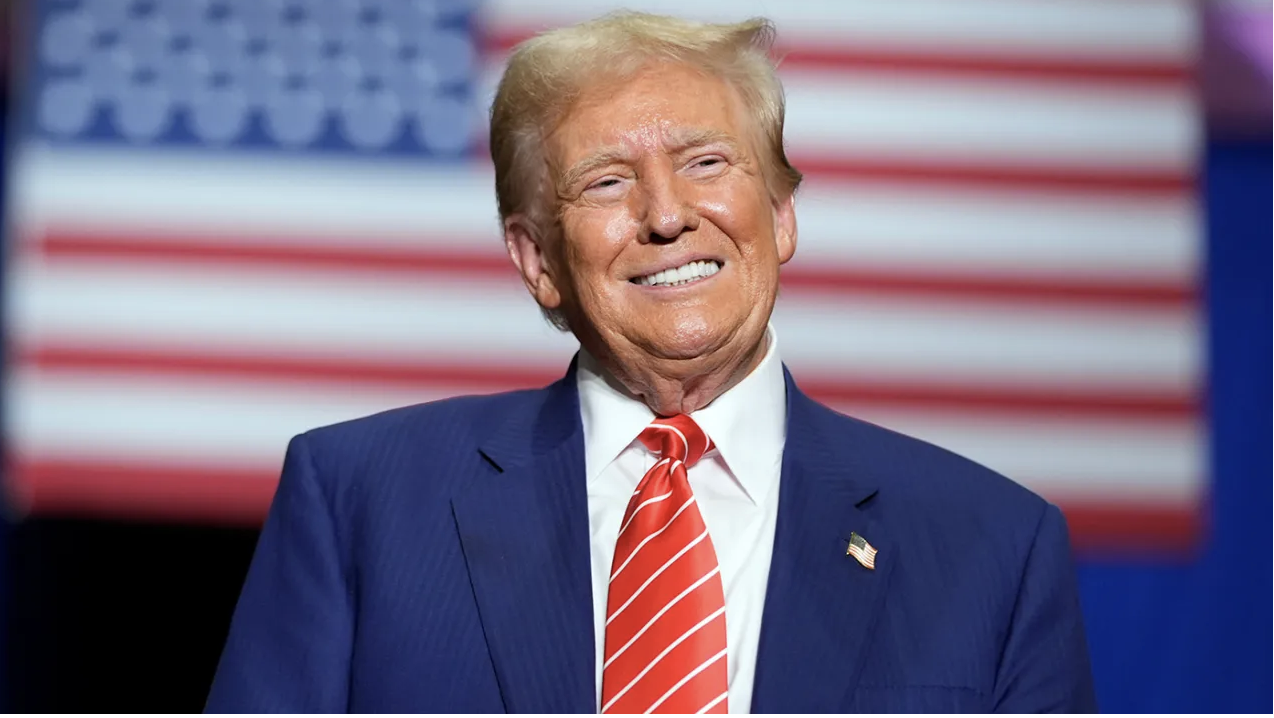
Featured Posts
-
Where To Watch Barcelona Vs Real Betis La Liga Match Time Tv Channels And Free Live Stream Links
May 16, 2025 -
Cassidy Hutchinson From Jan 6 Testimony To Tell All Memoir
May 16, 2025 -
Black Decker Steam Irons How To Choose The Best One For Your Needs
May 16, 2025 -
Goles Y Resumen Portugal Se Impone A Belgica 1 0
May 16, 2025 -
0 2 Olimpia Derrota A Penarol Resumen Goles Y Detalles Del Juego
May 16, 2025
Latest Posts
-
Muncy Speaks Out Addressing The Arenado Dodgers Trade Rumors
May 16, 2025 -
Is Hyeseong Kim The Solution Dodgers Recall Top Prospect To Mlb Roster
May 16, 2025 -
Dodgers Hyeseong Kim Fuels Doubleheader Sweep With Homer And Two Stolen Bases In Okc
May 16, 2025 -
Dodgers Recall Hyeseong Kim Analysis And Potential Lineup Changes
May 16, 2025 -
Oklahoma City Doubleheader Domination Hyeseong Kims Power Display For Dodgers
May 16, 2025