Google's Search AI: Continued Training Despite Opt-Outs
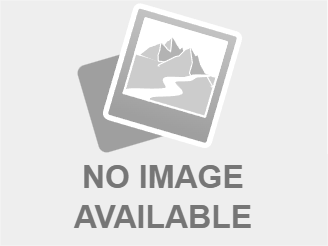
Table of Contents
How Google Uses User Data to Train its Search AI
Google's Search AI isn't powered by magic; it thrives on massive amounts of user data. Understanding how this data fuels the algorithm is crucial to grasping the privacy implications.
Data Sources for Google's AI Models
The dataset Google uses to train its AI models is colossal and diverse. It encompasses a wide range of information, including:
- Search Queries: Every search you conduct contributes to the training data, informing the algorithm about user intent and information needs.
- Browsing History: Your browsing habits, websites visited, and time spent on each site are valuable data points used to understand user behavior and preferences.
- Location Data: Your location, tracked through your device, helps Google personalize search results and understand local search trends.
- User Interactions: Clicks, dwell time on search results pages, and other user interactions provide crucial feedback on the relevance and quality of search results.
Anonymization Techniques and Their Limitations: Google employs various anonymization techniques to protect user identity. These include removing personally identifiable information (PII) and aggregating data. However, these methods aren't foolproof. There's a constant risk of re-identification, especially with large datasets.
- Federated Learning: Google utilizes federated learning, a technique that trains AI models on decentralized data without directly transferring the data to Google's servers. This method aims to improve privacy, but it still raises questions about the security and potential for indirect data leakage.
The Role of User Feedback in AI Improvement
User interactions with search results are integral to improving the AI's accuracy and relevance.
- Positive Aspects: User feedback directly influences the search algorithm's ranking system. Clicks on high-ranking results reinforce their relevance, while low engagement signals the need for improvement. This iterative process leads to increasingly relevant and personalized search experiences.
- Potential for Bias: The data used to train the AI reflects existing biases present in society. This can lead to biased search results, perpetuating stereotypes and inequalities. The algorithm learns from the data it's fed, meaning biased input results in biased output.
The Effectiveness of Google's Opt-Out Mechanisms
Google offers users several controls over their data, but the effectiveness of these controls in relation to Search AI training is debatable.
Understanding Google's Data Privacy Controls
Google provides options to limit data collection, such as:
- Web & App Activity: This setting controls the collection of your browsing and app usage data. Turning it off significantly reduces the data Google collects.
- Location History: Disabling Location History prevents Google from tracking your location.
- Personalized Ads: Opting out of personalized ads reduces the amount of data used for targeted advertising, although data may still be used for other purposes.
Nuances of Google's Policy: Even with opt-outs enabled, Google's privacy policy allows for the use of some anonymized data for AI training. The extent to which this data contributes to Search AI improvement is unclear, leading to transparency concerns.
The Debate on Data Minimization and Privacy
The debate centers on whether Google's massive dataset is truly necessary for AI development. Privacy advocates argue for data minimization – collecting only the data strictly necessary for a specific purpose.
- Privacy Advocates' Perspective: They highlight the risks associated with vast data collection, even with anonymization techniques, emphasizing the importance of user control and transparency. They advocate for stronger regulations and stricter adherence to privacy principles.
- Google's Perspective: Google defends its approach by highlighting the benefits of personalized search results and the advancements in AI made possible by large datasets. They argue that anonymization and federated learning mitigate privacy risks.
- GDPR and other Regulations: Regulations like the General Data Protection Regulation (GDPR) aim to strengthen data protection and user rights. Google’s practices are subject to scrutiny under such regulations.
The Long-Term Implications of Google's Approach
Google's data practices have far-reaching implications for users and the future of AI.
Impact on User Trust and Confidence
Google's continued use of user data for AI training, even after opt-outs, can erode user trust.
- Shift to Alternative Search Engines: Users concerned about privacy might switch to alternative search engines prioritizing user data protection. This could impact Google's market dominance.
- Erosion of Trust in AI: The lack of transparency and the perceived disregard for user privacy could damage public trust in AI-powered services more broadly.
Future of AI Development and Ethical Considerations
The ethical dilemmas surrounding AI development necessitate a broader conversation.
- Need for Stricter Regulations: Clearer regulations and stricter enforcement are needed to ensure responsible AI development that prioritizes user privacy.
- Transparent Data Practices: Greater transparency from companies about their data practices is crucial to building user trust and fostering ethical AI development.
- Privacy-Preserving AI Techniques: Investing in and developing privacy-preserving AI techniques is paramount to ensuring responsible innovation. This includes exploring differential privacy and homomorphic encryption.
Conclusion
Google's reliance on vast user data for its Search AI, coupled with the limitations of its opt-out mechanisms, raises serious ethical and privacy concerns. While Google highlights the benefits of personalized search results, the lack of complete transparency and the potential for re-identification remain significant challenges. Understanding these implications is crucial for users and the future of AI development. We encourage you to critically examine your own Google data settings and consider the implications of Google's approach to Google Search AI training. Explore your options for greater control over your data and perhaps investigate privacy-focused search engine alternatives. Learn more about protecting your online privacy and participating in the ongoing discussion about responsible AI development.
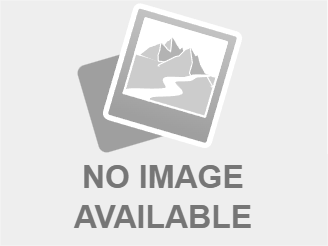
Featured Posts
-
Largest Heat Pump System Launched Innomotics Eneco And Johnson Controls Collaboration
May 04, 2025 -
Makron I S Sh A Novaya Strategiya Davleniya Na Rossiyu V Svyazi S Ukrainoy
May 04, 2025 -
Significant Shift Farage Overtakes Starmer In Uk Prime Ministerial Preference Poll
May 04, 2025 -
United Airlines Flight Cancellations In Newark The Faa Staff Walkout Fallout
May 04, 2025 -
1 T 50
May 04, 2025
Latest Posts
-
Singapore General Election A Test Of The Peoples Will
May 04, 2025 -
Singapores Political System Under Scrutiny The Upcoming Vote
May 04, 2025 -
The Stakes Are High Examining Singapores Upcoming Elections
May 04, 2025 -
Singapore Election Assessing The Political Landscape
May 04, 2025 -
Analyzing The Oppositions Chances In Singapores Next Election
May 04, 2025