How A Cybersecurity Expert Beat Deepfake Detection (CNN Business)
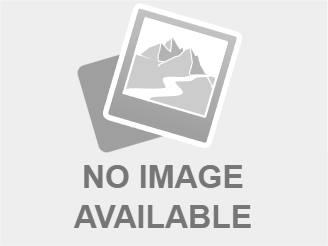
Table of Contents
The Expert's Methodology: Bypassing Deepfake Detection Systems
The cybersecurity expert employed a multi-pronged approach to bypass deepfake detection systems, exploiting subtle weaknesses in current detection algorithms. Their success underscores the need for continuous innovation in deepfake countermeasures. The methods used highlight the limitations of current technology and point towards future research directions.
- Employed subtle manipulation of existing deepfake algorithms, exploiting minor weaknesses. The expert didn't create entirely new deepfakes; instead, they manipulated existing ones, tweaking parameters to fall below the detection threshold of various algorithms. This suggests that even seemingly minor flaws in deepfake creation can be exploited.
- Utilized novel compression techniques to minimize detectable artifacts. Deepfakes often contain compression artifacts, telltale signs of digital manipulation. The expert employed advanced compression techniques, minimizing these artifacts and making the deepfake harder to detect. This involved sophisticated manipulation of image and video compression algorithms.
- Introduced imperceptible noise patterns to mask the deepfake's digital signature. Digital signatures, unique patterns embedded in deepfakes, are often used for detection. The expert introduced subtle noise patterns to disrupt these signatures, making them undetectable by existing algorithms. This involved introducing noise patterns that were imperceptible to the human eye but effectively masked the deepfake's unique digital fingerprint.
- Leveraged advanced adversarial machine learning to fool detection models. Adversarial machine learning focuses on creating inputs that deliberately mislead machine learning models. The expert used this technique to craft deepfakes that were specifically designed to evade detection by popular deepfake detection software. This technique essentially "trained" the deepfake to fool the detection systems.
- Focused on exploiting specific limitations within the target detection software. The expert's approach wasn't generalized; they identified and exploited specific weaknesses in the algorithms of popular deepfake detection software. This highlights the crucial importance of continuous updates and improvements within detection software.
This detailed approach showcases how even seemingly minor flaws in current deepfake detection methods can be leveraged to create undetectable deepfakes. The ability to counteract common detection methods such as analyzing facial inconsistencies, flickering pixels, and inconsistencies in metadata highlights the ongoing need for more robust detection strategies.
The Implications for Cybersecurity and National Security
The successful circumvention of deepfake detection systems has significant implications for cybersecurity and national security. The potential for misuse is vast, and the consequences can be severe.
- Increased risk of disinformation and propaganda campaigns. Deepfakes can be used to create convincing fake videos or audio recordings that spread misinformation and manipulate public opinion. This poses a severe threat to democratic processes and societal stability.
- Potential for identity theft and financial fraud. Deepfakes can be used to impersonate individuals to gain access to sensitive information or commit financial crimes. This includes impersonating bank officials or other trusted figures.
- Threats to national security through manipulated evidence or impersonation. Deepfakes can be used to create false evidence, implicate innocent individuals, or impersonate government officials, undermining national security. This risk extends to both political and military contexts.
- Erosion of public trust in media and information sources. The prevalence of undetectable deepfakes could erode public trust in all forms of media, leading to widespread skepticism and making it harder to discern truth from falsehood.
- Challenges to the legal system regarding evidence authenticity. The ability to create highly convincing deepfakes poses significant challenges to the legal system, complicating the process of determining evidence authenticity in criminal investigations and legal proceedings.
The Future of Deepfake Detection: An Ongoing Arms Race
The expert's success underscores the critical need for continuous innovation in deepfake detection technology. This is an ongoing arms race, requiring a collaborative approach.
- The need for continuous improvement and innovation in deepfake detection technology. We need faster, more adaptive, and more robust detection algorithms capable of handling increasingly sophisticated deepfakes.
- Development of more robust and adaptive detection algorithms. Future detection methods will need to be more adaptable and capable of handling the constant evolution of deepfake creation techniques.
- Collaboration between researchers, cybersecurity experts, and technology companies. A collaborative effort is essential to tackle this complex problem, requiring the combined expertise of different fields.
- Exploring new methods of identifying deepfakes through advanced analysis techniques. Researchers are exploring advanced techniques, including those based on blockchain and watermarking, to create more resilient deepfake detection methods.
- The importance of media literacy and critical thinking in identifying potentially fake content. Individuals also need to develop critical thinking skills and media literacy to better identify potentially fake content, regardless of technological solutions.
Conclusion
This article highlighted how a cybersecurity expert successfully circumvented existing deepfake detection systems, revealing critical vulnerabilities in current technology. The implications for cybersecurity, national security, and public trust are significant, demanding a proactive response from researchers, policymakers, and individuals alike. The ability to defeat deepfake detection underscores the urgent need for continued research and development in this field. Stay informed about the latest advancements in deepfake detection and prevention strategies to protect yourself from the ever-evolving threats of deepfake technology. Learn more about deepfake detection and how to identify potential deepfakes to safeguard yourself and contribute to a more informed and secure digital world.
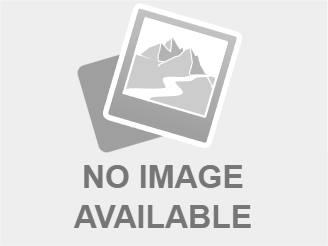
Featured Posts
-
Tam Krwz Ke Jwtwn Pr Mdah Ka Hmlh Swshl Mydya Pr Rdeml Ky Lhr
May 17, 2025 -
Is La Ligas Anti Piracy Strategy Unacceptable Censorship Vercel Weighs In
May 17, 2025 -
Ohio Train Derailment Aftermath Persistent Toxic Chemical Contamination In Structures
May 17, 2025 -
Will Trumps China Tariffs Remain At 30 Until Late 2025
May 17, 2025 -
Wnba Star Accuses Angel Reese Of Tampering
May 17, 2025
Latest Posts
-
Week In Review Reflecting On Past Mistakes
May 17, 2025 -
Mlb Injury News Mariners Bryce Miller To 15 Day Il Elbow
May 17, 2025 -
How To Spot And Avoid Sharing Fake Angel Reese Quotes
May 17, 2025 -
Analyzing The Spread Of Misinformation The Case Of Angel Reese
May 17, 2025 -
Bryce Miller Mariners On 15 Day Il Elbow Injury Update
May 17, 2025