How Google Trains Its Search AI: Even After Opting Out
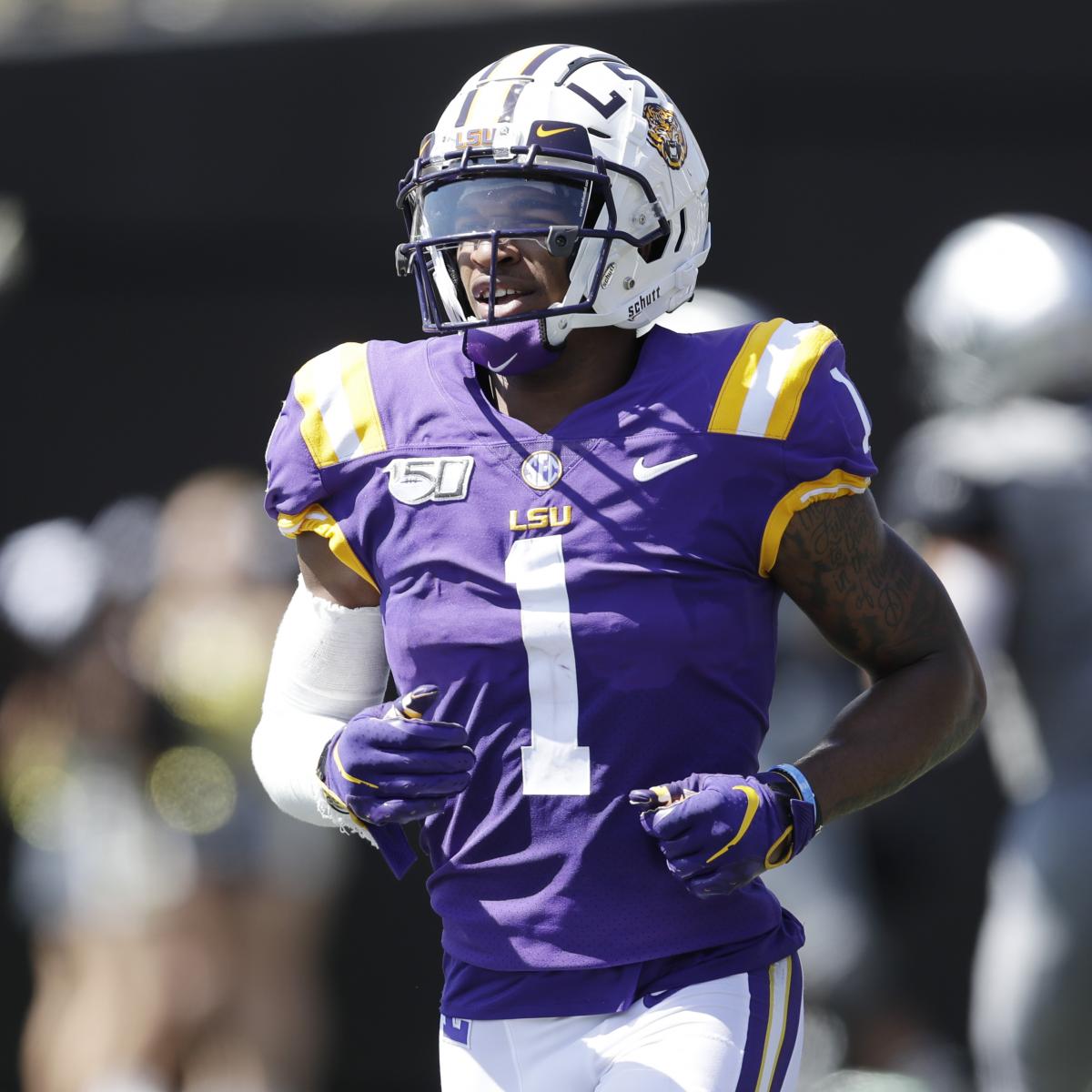
Table of Contents
Data Collection Practices Even After Opting Out
Google's commitment to improving its search engine relies heavily on data. Even with opt-out selections, various data streams contribute to the ongoing training of its AI.
Anonymization Techniques
Google employs various anonymization techniques to protect user privacy while still leveraging data for AI training. However, the effectiveness of these techniques is a subject of ongoing debate.
- Removing Personally Identifiable Information (PII): This involves stripping away details like names, addresses, and phone numbers.
- Data Aggregation: Combining data from numerous users to create aggregate statistics, obscuring individual contributions.
- Differential Privacy: Adding carefully calibrated noise to data to prevent the re-identification of individuals.
Despite these measures, research suggests that re-identification is sometimes possible, even with sophisticated anonymization techniques. For instance, studies have shown that seemingly anonymized datasets can be linked to specific individuals using external information sources. This highlights the inherent limitations of data anonymization. The ongoing discussion surrounding data anonymization and its effectiveness is crucial in the context of Google data privacy and user data protection.
Publicly Available Data
Google also leverages vast quantities of publicly available data to train its AI. This includes information freely accessible on the internet.
- Publicly indexed websites: The content of billions of web pages forms a critical component of Google's training data.
- Open-source datasets: Publicly available datasets on topics ranging from language translation to image recognition contribute to model refinement.
- Government data: Where legally accessible and ethically permissible, publicly released government data may also inform AI training.
This public data contributes significantly to the AI's understanding of language, facts, and relationships between different concepts, thereby enhancing search relevance and accuracy. The process of web crawling plays a key role in collecting this open-source data.
Implicit Data Collection
Even with explicit opt-outs, Google implicitly collects data through user interactions with its services.
- Search queries: The terms you search for provide invaluable insights into user intent and information needs.
- Clickstream data: The sequence of links you click on reveals your navigation patterns and interests.
- Location data (even when location services are off): Approximate location data can be inferred from IP addresses and other signals.
This implicit data raises ethical concerns regarding user consent and privacy. Understanding the nature of behavioral data and user interaction data is crucial for informed consent.
The Role of User Data in AI Training
User data plays a crucial role in the training and improvement of Google's search AI, particularly through machine learning techniques.
Supervised Learning and Unsupervised Learning
Google employs both supervised and unsupervised learning methods in its AI development.
- Supervised Learning: This involves training the AI on labeled data, where the desired outcome is known. For example, Google might train its algorithm on a dataset of search queries paired with the user's subsequent clicks, allowing the AI to learn which results are most relevant.
- Unsupervised Learning: This involves training the AI on unlabeled data, allowing it to discover patterns and relationships without explicit guidance. For example, Google might use unsupervised learning to identify clusters of similar search queries.
These AI algorithms benefit greatly from the vast quantities of user data, enabling both the refinement of existing models and the development of new functionalities. Machine learning and deep learning are pivotal to these processes.
Improving Search Relevance and Accuracy
User data directly impacts search result relevance and accuracy.
- Ranking algorithms: User clicks, dwell time (how long a user stays on a page), and bounce rate (how quickly a user leaves a page) all influence the ranking of search results.
- Personalized search: User data allows Google to tailor search results to individual preferences and past behavior.
Essentially, user feedback, captured through various metrics such as clicks and dwell time, directly informs the AI's learning process, continuously refining its ability to deliver the most relevant results. This continuous improvement loop is critical for search engine optimization and optimizing the search algorithm. The goal is to provide the most relevant personalized search results.
Transparency and User Control
While Google strives for transparency, the complexities of AI training limit user control.
Google's Transparency Efforts
Google makes efforts to be transparent about its data practices.
- Google's Privacy Policy: This extensive document details data collection and usage practices.
- Data tools and settings: Users can access and manage various aspects of their data through their Google accounts.
However, the sheer volume and complexity of data processing make complete comprehension difficult, limiting the effectiveness of these transparency efforts.
Limitations of User Control
Even with opt-out options, complete control over data used for AI training remains limited.
- Data anonymization limitations: As discussed, anonymization is not foolproof.
- Implicit data collection: Much data is collected implicitly, often without explicit user awareness.
This highlights the need for proactive strategies from users, such as data minimization and a heightened awareness of online privacy. Understanding your data privacy control options and employing them effectively are crucial steps to mitigating potential risks.
Conclusion
Even after opting out of certain data collection practices, Google still utilizes anonymized and publicly available data, along with implicit data, to train its search AI, leading to improvements in search relevance and accuracy. Understanding how Google trains its search AI is crucial for navigating the digital landscape. Take control of your privacy settings, but also be aware of the inherent limitations. Stay informed about data privacy and AI development to make informed decisions about your online presence.
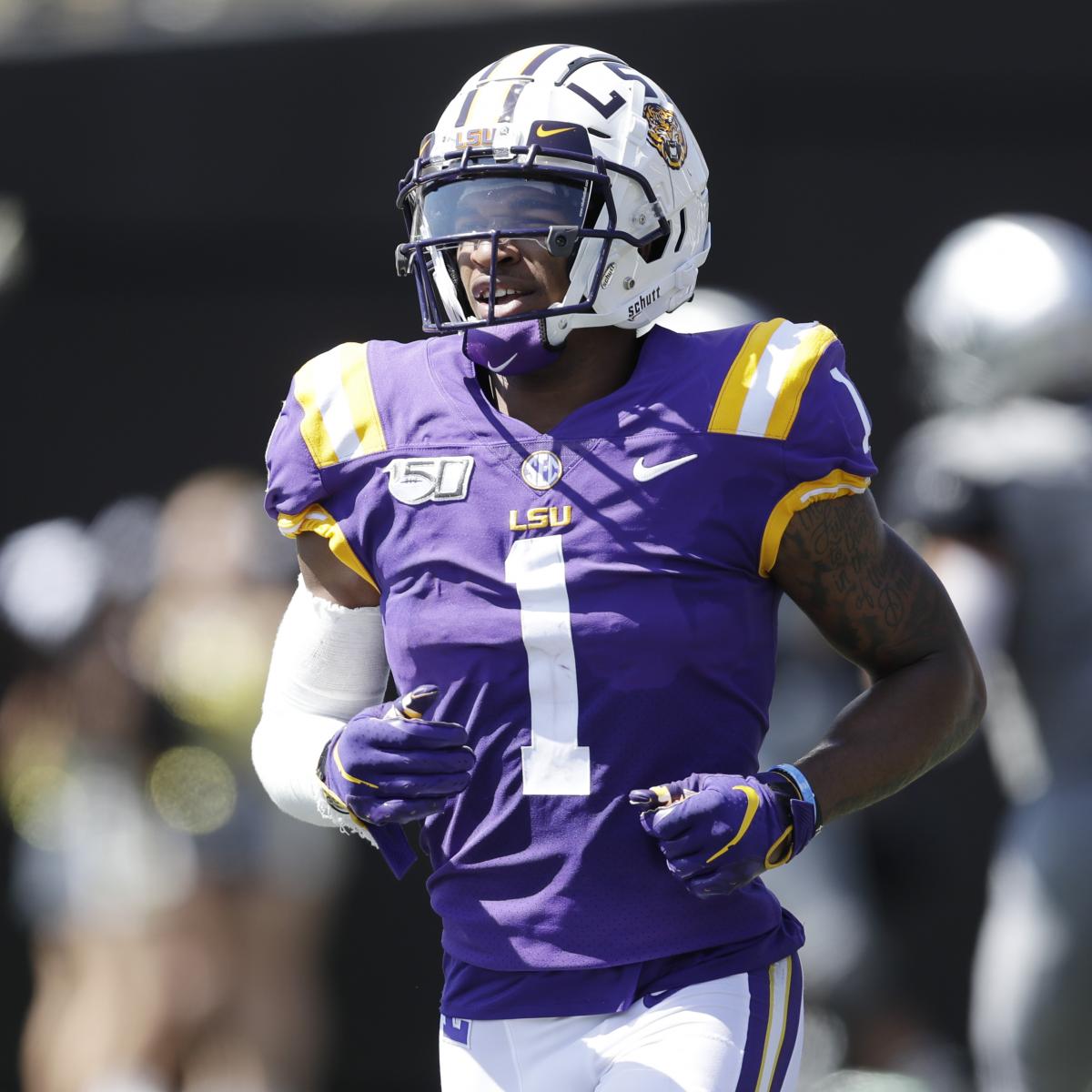
Featured Posts
-
Expensive Offshore Wind Farms Why Energy Firms Are Hesitant
May 04, 2025 -
Farages New Slogan A Controversial Jimmy Savile Reference
May 04, 2025 -
Seven Dead After Truck And Van Collision Near Yellowstone National Park
May 04, 2025 -
Ice Evasion Migrant Remains Hidden In Tree For Eight Hours
May 04, 2025 -
270 M Wh Bess Financing In Belgium A Comprehensive Overview
May 04, 2025
Latest Posts
-
Rare Novel Valued At 45 000 Discovered In Bookstore
May 04, 2025 -
45 000 Rare Book A Bookstores Unexpected Find
May 04, 2025 -
Singapore General Election A Test Of The Peoples Will
May 04, 2025 -
Singapores Political System Under Scrutiny The Upcoming Vote
May 04, 2025 -
The Stakes Are High Examining Singapores Upcoming Elections
May 04, 2025