How Outdated Business Apps Hamper AI Implementation
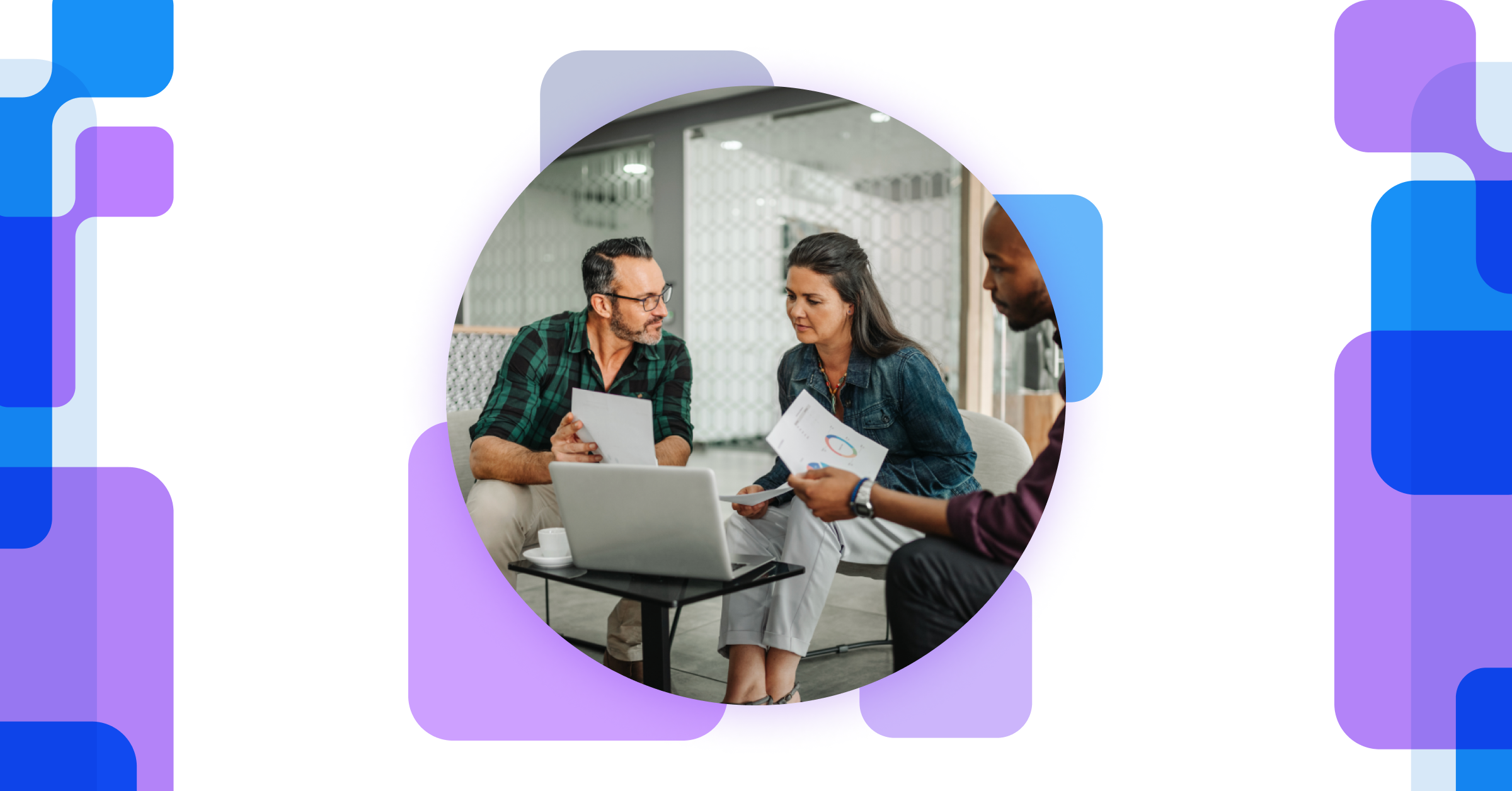
Table of Contents
Incompatibility Issues with Modern AI Tools
Outdated business apps frequently clash with the demands of modern AI tools, creating significant roadblocks to integration. These incompatibility issues stem from several core problems.
Data Format Conflicts
Legacy systems often rely on outdated data formats that are simply incompatible with modern AI algorithms. This creates a significant hurdle to effective AI implementation.
- Examples of incompatible formats: Many older systems use legacy databases with proprietary formats, making data extraction and migration difficult. Incompatible APIs further complicate matters, hindering seamless data flow.
- Challenges in data conversion: Converting data from these legacy formats to formats suitable for AI often requires significant manual effort, specialized tools, and substantial time investments.
- Time & resource costs: The costs associated with data migration and cleansing can be substantial, delaying AI projects and consuming valuable resources that could be directed towards AI development and deployment. This often necessitates expertise in data migration and API integration, adding to the expense.
Lack of Scalability and Flexibility
Outdated apps often lack the scalability and flexibility required for AI. Modern AI requires substantial computing power and large datasets – capabilities often absent in legacy systems.
- Examples of limitations: Slow processing speeds, limited storage capacity, and inflexible architectures often prevent AI models from being trained and deployed effectively.
- Implications for AI model training and deployment: Training complex AI models demands significant computing resources. Limited capacity prevents the use of advanced algorithms and limits the accuracy and capabilities of AI models.
- Need for scalable infrastructure: A shift to cloud computing and the adoption of scalable infrastructure are often necessary to overcome these limitations, adding another layer of complexity and cost to the transition. This requires careful consideration of big data analytics and machine learning infrastructure.
Data Integration Challenges and Siloed Data
Perhaps the most significant obstacle presented by outdated business apps is the challenge of data integration. Many organizations grapple with data trapped in disparate legacy systems, creating significant hurdles for AI implementation.
Data Silos and Lack of Centralized Data Management
Data silos – separate systems holding critical information – are common in organizations reliant on legacy systems. This fragmented data landscape makes it difficult to build a holistic view necessary for effective AI.
- Examples of data silos: CRM, ERP, marketing automation tools, and other legacy applications often operate independently, storing data in isolated locations.
- Difficulties in consolidating data for analysis: Combining data from various sources is a complex process, requiring sophisticated data integration techniques and specialized expertise.
- Impact on AI model accuracy: Inaccurate or incomplete datasets, resulting from data silos, dramatically affect the accuracy and reliability of AI models. Effective data governance and data warehousing strategies are essential.
Difficulty in Data Access and Sharing
Access and sharing data across different systems often pose significant challenges. This impacts AI training, real-time insights, and collaborative efforts.
- Examples of access restrictions: Legacy systems often have complex security protocols that hinder data access. Lack of interoperability prevents seamless data exchange between different systems.
- Impact on collaboration and AI-driven decision making: Restricted data access hampers collaboration among teams and limits the ability to derive valuable insights from data for effective decision-making.
- Importance of API management and data sharing: Implementing robust API management systems and establishing clear data sharing policies are crucial to overcome these limitations. Maintaining data security and data privacy is paramount.
Security Risks Associated with Outdated Business Apps
Outdated business apps often present significant security risks, jeopardizing sensitive data vital for AI applications.
Vulnerability to Cyber Threats
Legacy systems frequently lack the advanced security features found in modern applications, making them vulnerable to cyber threats.
- Examples of security vulnerabilities: Outdated security protocols, insufficient encryption, and lack of patching leave systems susceptible to data breaches.
- Risks of data breaches: Data breaches not only lead to financial losses but can also severely damage an organization's reputation and lead to legal ramifications.
- Compliance issues: Failure to meet data security standards and compliance regulations can result in hefty fines and legal action. Robust risk management strategies are critical.
Difficulty in Maintaining Data Integrity
Outdated apps often struggle to maintain data integrity, leading to inconsistencies and errors. This negatively impacts AI model accuracy.
- Examples of data corruption, inconsistencies, and inaccuracies: Data corruption, human error, and system malfunctions can introduce inaccuracies and inconsistencies in data stored in legacy systems.
- Impact on AI model training and decision making: AI models trained on inaccurate data yield unreliable results and lead to flawed decisions. Data quality management is critical.
- Importance of data validation and error correction: Implementing robust data validation and error correction mechanisms is crucial for maintaining data integrity and ensuring the reliability of AI models.
The High Cost of Upgrading and the ROI of Modernization
While upgrading legacy systems involves upfront costs, the long-term benefits of modernization far outweigh the initial investment.
Financial Investment Required for Modernization
Modernizing IT infrastructure necessitates a significant financial investment. This encompasses software licensing, hardware upgrades, and professional services.
- Breakdown of potential costs: Costs include purchasing new software licenses, upgrading hardware (servers, storage), and hiring consultants or internal IT staff for implementation and training.
- Financing options: Organizations can explore various financing options such as leasing, subscription models, and phased implementations to manage the financial burden of modernization.
- Phased approach to modernization: A phased approach, upgrading systems gradually rather than all at once, can help manage costs and minimize disruption.
Long-Term Benefits of Modernizing for AI
Modernizing IT infrastructure provides significant long-term financial benefits by enabling efficient AI integration.
- Improved efficiency: Streamlined processes and automated workflows enhance operational efficiency, reducing costs and increasing productivity.
- Increased productivity: AI-driven automation frees up human resources to focus on higher-value tasks, leading to increased productivity.
- Better data insights: Access to a unified, reliable dataset enables organizations to gain better insights, leading to improved decision-making.
- Enhanced decision-making: AI-driven analytics provides data-driven insights, improving the quality of decisions made across the organization.
- Competitive advantage: Embracing AI-driven modernization helps businesses gain a significant competitive advantage in their respective markets. A strong digital transformation strategy is essential.
Conclusion
Outdated business apps significantly hamper AI implementation, creating incompatibility issues, data integration challenges, security risks, and substantial upgrade costs. Ignoring these challenges can lead to significant setbacks in leveraging the full potential of AI. Don't let outdated business apps hamper your AI implementation. Assess your current IT infrastructure and consider a strategic modernization plan. Start your modernization journey today! Failing to embrace AI-driven modernization puts your business at a significant competitive disadvantage in today's rapidly evolving digital landscape. The return on investment from modernizing your systems to support AI is substantial and crucial for long-term success.
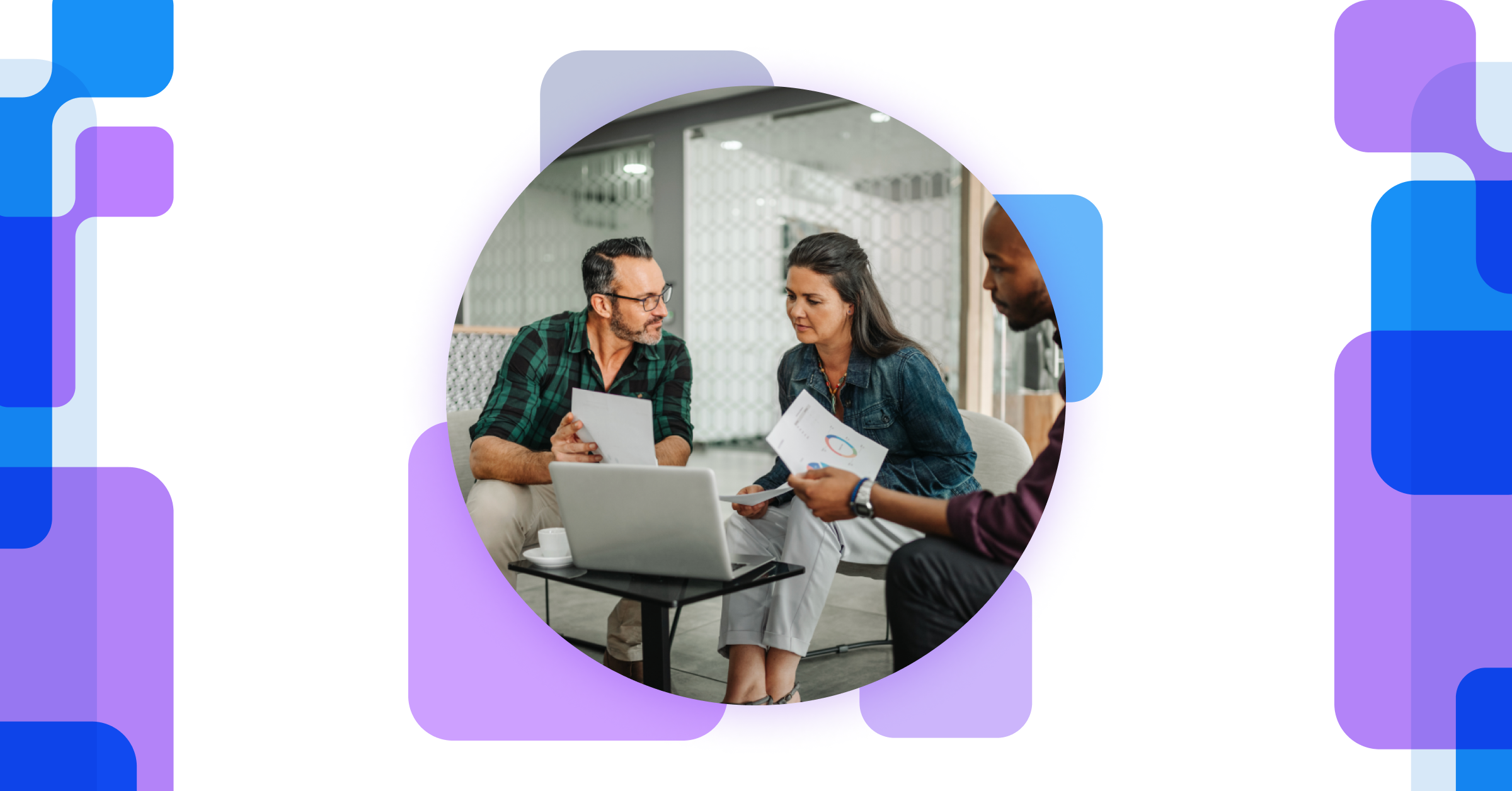
Featured Posts
-
Trumps Approval Rating Plummets A Deep Dive Into The First 100 Days
Apr 30, 2025 -
Chilean Migrants And The Nfl Heists A Multi Million Dollar Crime Spree
Apr 30, 2025 -
Argamani At Time Gala Demands For Hostage Release In Israel
Apr 30, 2025 -
Broadcoms V Mware Deal Concerns Over Extreme Price Increases For Customers
Apr 30, 2025 -
Sedlacek Predvida Jokic I Jovic Na Evrobasketu
Apr 30, 2025
Latest Posts
-
Charlotte Jordans Coronation Street Exit First Look At Daisy Midgeleys Farewell
May 01, 2025 -
Old Lantern Barn In Charlotte Ownership Opportunity From Farmers And Foragers
May 01, 2025 -
Watch Live Dr Victoria Watlingtons Town Hall On Wsoc Tv With Joe Bruno
May 01, 2025 -
Nfl Draft Panthers 8th Pick Holds High Expectations
May 01, 2025 -
Daisy Midgeleys Coronation Street Departure Charlotte Jordans Final Scenes
May 01, 2025