Is Your Legacy Software Holding Back Your AI Vision?
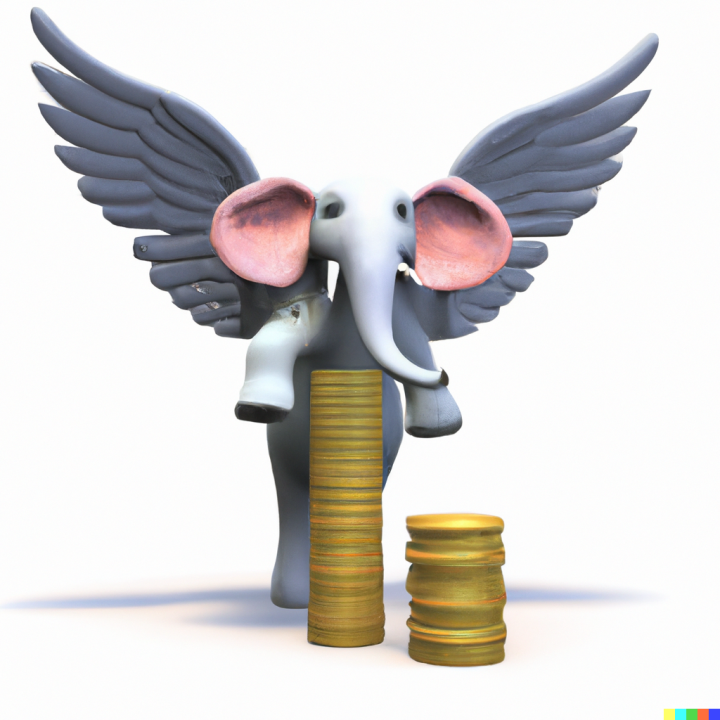
Table of Contents
The Incompatibility Challenge: Why Legacy Systems and AI Clash
Integrating AI into a business requires seamless data flow, scalable infrastructure, and robust security. Legacy systems, often characterized by outdated technology and fragmented architectures, frequently fall short in these areas. This incompatibility creates significant hurdles in realizing the full potential of AI.
Data Silos and Integration Difficulties
One of the most significant challenges posed by legacy systems is the problem of data silos. Data is often scattered across various incompatible systems, making it incredibly difficult to aggregate and prepare for AI/ML model training. This results in incomplete datasets, hindering the accuracy and effectiveness of AI algorithms.
- Lack of APIs: Many legacy systems lack the application programming interfaces (APIs) necessary for seamless data exchange with modern AI platforms.
- Incompatible data formats: Data may be stored in various outdated and incompatible formats, requiring extensive data transformation before it can be used by AI models.
- Difficulty in data extraction and cleaning: Extracting and cleaning data from legacy systems can be a time-consuming and complex process, demanding significant resources and expertise. This is a major bottleneck in legacy system data integration for AI projects. The resulting data silo problem directly impacts the quality of AI insights. Ensuring AI data compatibility is crucial for success.
Scalability and Performance Bottlenecks
Legacy systems often lack the scalability and performance needed to support the demands of modern AI algorithms and large datasets. AI models require significant computing power and storage capacity, which older systems may not be able to provide.
- Slow processing speeds: The slow processing speed of legacy systems can significantly delay AI model training and inference, hindering real-time applications.
- Limited storage capacity: Legacy systems may not have the storage capacity to accommodate the large datasets required for effective AI model training.
- Inability to handle real-time data streams: Many AI applications require the ability to process real-time data streams, a capability that many legacy systems lack. This is a key consideration for legacy system scalability and AI performance bottlenecks. Effective real-time data processing is critical for many AI applications.
Security Risks and Compliance Issues
Integrating AI into outdated systems with inadequate security measures introduces significant security risks and compliance challenges. Legacy systems are often vulnerable to cyber threats and may not meet modern data protection standards.
- Vulnerabilities in legacy code: Outdated code in legacy systems may contain vulnerabilities that can be exploited by attackers, compromising sensitive data used by AI models.
- Lack of data encryption: Legacy systems may lack adequate data encryption, increasing the risk of data breaches.
- Difficulty meeting regulatory compliance requirements: Integrating AI into legacy systems can create challenges in meeting various regulatory compliance requirements, such as GDPR and HIPAA. Addressing legacy system security and ensuring AI data security are paramount, as is compliance with AI regulations.
Overcoming the Hurdles: Strategies for Successful AI Integration
Addressing the challenges posed by legacy systems requires a strategic approach to modernization and data integration. This involves a combination of technological upgrades and careful planning.
Modernization Strategies
Modernizing legacy systems is a crucial step in enabling successful AI integration. This involves various approaches, each tailored to specific circumstances.
- Cloud-native applications: Migrating legacy applications to the cloud provides scalability, flexibility, and improved security.
- Microservices architecture: Breaking down monolithic legacy applications into smaller, independent microservices improves agility and facilitates incremental upgrades.
- API gateways: Implementing API gateways provides a controlled and secure way to access data from legacy systems, allowing seamless integration with modern AI platforms. These strategies are critical components of legacy system modernization and are crucial for implementing cloud migration for AI and leveraging a microservices architecture.
Data Integration and Management Solutions
Effective data integration is critical for successful AI adoption. This requires tools and techniques to consolidate and prepare data from disparate sources.
- ETL (Extract, Transform, Load) processes: ETL processes are essential for extracting data from legacy systems, transforming it into a usable format, and loading it into a data warehouse or data lake.
- Data lakes: Data lakes provide a centralized repository for storing raw data from various sources, including legacy systems, enabling efficient data analysis and AI model training.
- Data virtualization: Data virtualization provides a unified view of data from multiple sources, including legacy systems, without requiring physical data migration. These tools are key for effective data integration for AI, enabling optimal data lake implementation, and streamlining ETL processes for legacy systems.
Choosing the Right AI Partner
Selecting a reliable partner with expertise in integrating AI with legacy systems is crucial for successful implementation.
- Experience with legacy system upgrades: Look for partners with a proven track record of successfully upgrading and modernizing legacy systems.
- Data migration expertise: Ensure your partner has experience migrating data from legacy systems to modern AI platforms.
- AI model deployment skills: Choose a partner with expertise in deploying and managing AI models in production environments. Partner selection is vital; therefore, finding the right AI integration partners, especially those with experience as legacy system consultants, is essential for securing the best AI implementation services.
Conclusion
Legacy software presents significant challenges to AI adoption, creating obstacles related to data integration, scalability, security, and compliance. However, by implementing modernization strategies, adopting robust data integration solutions, and choosing the right AI partner, businesses can overcome these hurdles. The key takeaways are that seamless data integration for AI is paramount; proactive legacy system modernization is key; and choosing the right AI integration partners is essential. Don't let your legacy software hold back your AI vision. Assess your current systems and explore modernization strategies today to unlock the full potential of AI for your business. Investing in overcoming the challenges presented by legacy systems is an investment in a future powered by effective AI.
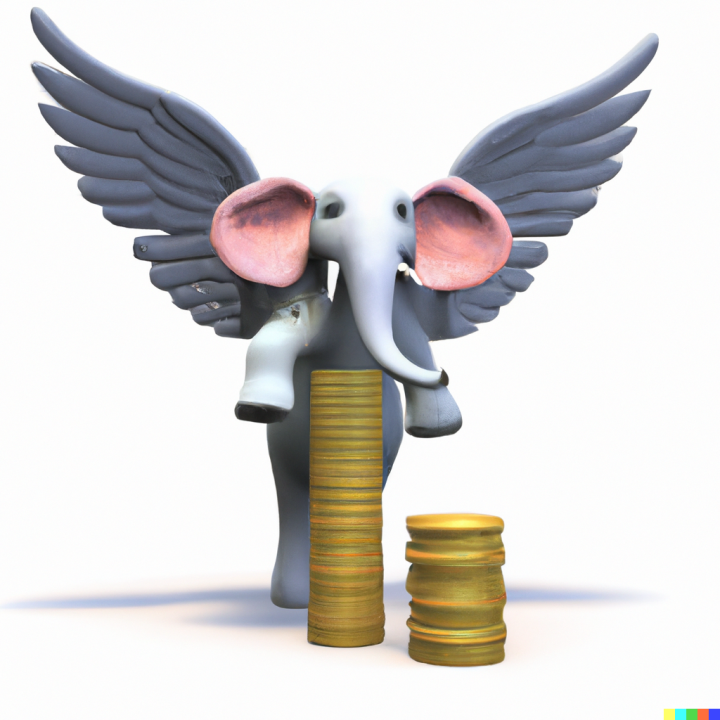
Featured Posts
-
Post Election Analysis Assessing Poilievres Defeat In Canada
Apr 30, 2025 -
Dai Su Tinh Nguyen Tien Linh Gop Phan Xay Dung Binh Duong Van Minh
Apr 30, 2025 -
No Te Lo Pierdas Clases De Boxeo Edomex Solo 3 Dias
Apr 30, 2025 -
Data Privacy Case France Fines Apple E162 Million
Apr 30, 2025 -
Pro Bono Deal Prevents Sanctions For Fourth Firm Working With Trump
Apr 30, 2025
Latest Posts
-
Gia Tieu Tang Tin Vui Cho Nong Dan Viet Nam
May 01, 2025 -
Essential Michael Jordan Facts A Concise Overview
May 01, 2025 -
Thi Truong Tieu Hom Nay Gia Tang Co Hoi Cho Nong Dan
May 01, 2025 -
Target Starbucks Vs Standalone 9 Key Differences
May 01, 2025 -
Gia Tieu Hom Nay Phan Tich Thi Truong Va Trien Vong Cho Nong Dan
May 01, 2025