Legacy Systems And AI: A Recipe For Disaster
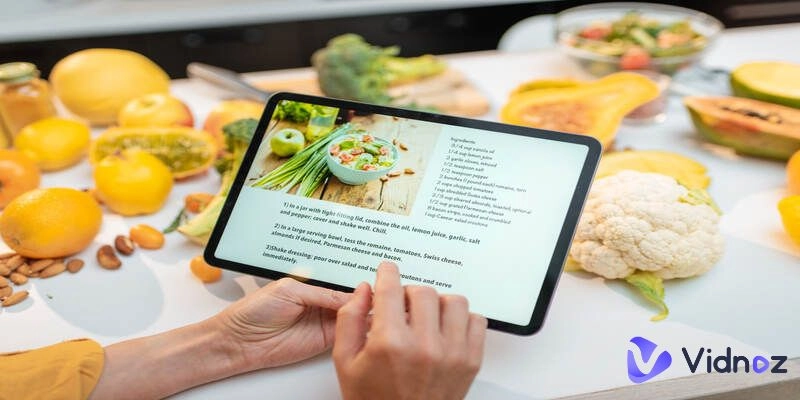
Table of Contents
Data Incompatibility and Integration Challenges
One of the most significant hurdles in integrating AI with legacy systems is data incompatibility. Extracting, transforming, and loading (ETL) data from these systems into AI-compatible formats is often a complex and time-consuming process. Many legacy systems rely on outdated data formats like COBOL or store data in flat files, making it difficult to integrate seamlessly with modern AI platforms that typically require structured, relational databases. Furthermore, data silos are common in organizations with legacy systems, hindering interoperability and creating significant challenges for AI model training. The lack of standardized APIs or interfaces further complicates data access.
- Different data formats: Legacy systems often use archaic formats (e.g., COBOL, flat files) incompatible with modern AI tools.
- Data quality issues: Inconsistent data, errors, and missing values significantly impact AI model accuracy and reliability. Cleaning and preparing this data can be incredibly resource-intensive.
- Lack of APIs/interfaces: The absence of Application Programming Interfaces (APIs) or standardized interfaces makes accessing and integrating data incredibly challenging.
- High cost and time of data migration: Migrating data from legacy systems is often costly and time-consuming, requiring specialized expertise and significant investment.
Security Risks and Vulnerabilities
Integrating AI with legacy systems significantly expands the attack surface, increasing the organization's vulnerability to cyber threats. Outdated systems often lack robust security features like encryption, access controls, and regular security patching. This makes them prime targets for cyberattacks, potentially leading to data breaches, financial losses, and reputational damage. The complexity of integrating AI components with legacy systems can also introduce new vulnerabilities if not handled correctly.
- Lack of robust security features: Many legacy systems were developed before modern security best practices became commonplace, increasing the risk of exploitation.
- Outdated software: Older software versions often contain known vulnerabilities that are readily exploited by malicious actors.
- Potential for data breaches: A successful breach can lead to significant financial losses, legal liabilities, and damage to the organization's reputation.
- Compliance issues: Failure to meet regulatory requirements like GDPR and CCPA can result in substantial fines and penalties.
Scalability and Performance Issues
Legacy systems, often built on older infrastructure, may struggle to handle the increased data volume and processing demands of AI applications. Performance bottlenecks are common, leading to slow processing speeds and inefficiencies. Scaling the infrastructure to meet the growing computational requirements of AI workloads can also prove problematic and costly. The limitations of legacy hardware and software can severely hamper the performance of AI models, hindering their effectiveness.
- Limited computing power: Legacy systems may lack the processing power necessary for computationally intensive AI tasks.
- Slow processing speeds: Slow processing times can lead to delays, reduce efficiency, and impact overall productivity.
- Difficulty scaling infrastructure: Expanding the infrastructure to support AI workloads can be challenging and expensive with legacy systems.
- Increased maintenance costs: Maintaining both legacy and new AI infrastructure increases operational costs and complexity.
The Cost of Failure
The consequences of poorly planned AI integration with legacy systems can be devastating. Financial losses due to system downtime, data breaches, and project overruns can reach millions of dollars. Reputational damage resulting from security incidents or failed AI initiatives can severely impact an organization's standing. Furthermore, legal ramifications, including fines for non-compliance with data protection regulations, add to the significant cost of failure. For instance, a major retailer experiencing a data breach due to legacy system vulnerabilities could face millions in fines and lost customer trust.
Conclusion
Integrating AI with legacy systems presents a multitude of challenges, including data incompatibility, heightened security risks, scalability issues, and the substantial cost of failure. Successfully navigating these challenges requires careful planning, a phased approach, and robust mitigation strategies. This includes developing comprehensive data migration strategies, implementing rigorous security measures, and selecting AI solutions compatible with existing infrastructure. Don't let your legacy systems become a roadblock to AI adoption. Plan your integration strategy carefully to avoid the pitfalls of legacy systems and AI incompatibility. Contact us today to learn more about mitigating the risks and harnessing the power of AI effectively.
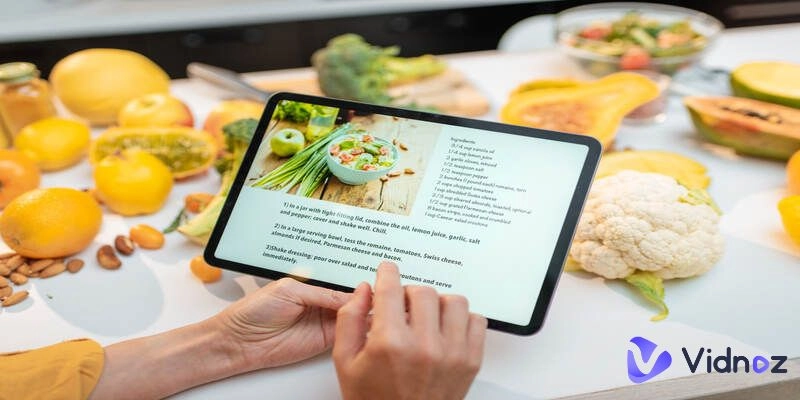
Featured Posts
-
Cau Thu Tien Linh Su Cong Hien Tham Lang Cho Cong Dong Binh Duong
Apr 30, 2025 -
Bbc Panorama And Chris Kaba The Iopcs Ofcom Action
Apr 30, 2025 -
Time Magazines 100 Most Influential The Story Of Noa Argamani
Apr 30, 2025 -
Fdyht Shbab Bn Jryr Ryys Alnady Yudan Qdayya
Apr 30, 2025 -
Processo Becciu Data Appello 22 Settembre E Dichiarazione Di Innocenza
Apr 30, 2025
Latest Posts
-
Will The Panthers 8th Pick Deliver Another Star
May 01, 2025 -
Coronation Street Daisy Midgeleys Exit Story Revealed
May 01, 2025 -
Farmers And Foragers Charlottes Old Lantern Barn Available For Purchase
May 01, 2025 -
Panthers 8th Overall Pick A Crucial Selection For Continued Success
May 01, 2025 -
Charlotte Jordans Coronation Street Exit First Look At Daisy Midgeleys Farewell
May 01, 2025