Quantum AI In Drug Discovery: D-Wave's (QBTS) Contributions And Future Potential
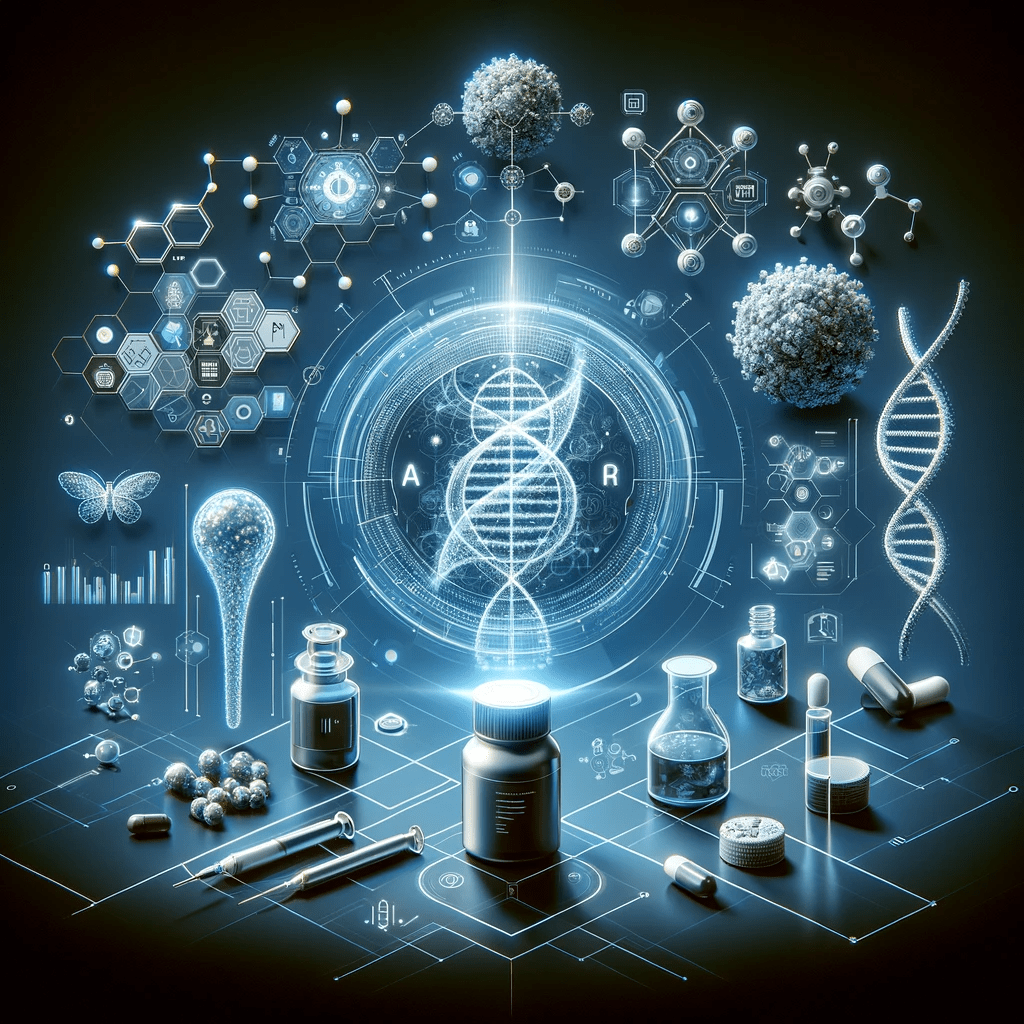
Table of Contents
D-Wave's (QBTS) Quantum Annealing Approach to Drug Discovery
Traditional methods in drug design often struggle with the immense complexity of molecular interactions. Simulating these interactions accurately requires immense computational power, often exceeding the capabilities of even the most powerful classical computers. This is where quantum annealing, the technology at the heart of D-Wave's quantum computers, steps in.
Addressing Computational Challenges in Drug Design
Classical computing approaches face significant hurdles in drug design, including:
- Protein folding: Predicting the three-dimensional structure of proteins is crucial for understanding their function and designing drugs that interact with them. This is a notoriously difficult computational problem.
- Ligand docking: Determining how well a potential drug molecule (ligand) binds to a target protein is essential for assessing its efficacy. Traditional methods can be computationally expensive and time-consuming.
- Virtual screening: Evaluating vast libraries of potential drug candidates to identify promising molecules is a massive computational task.
Quantum annealing offers a potential solution by leveraging the principles of quantum mechanics to explore the energy landscape of molecular interactions far more efficiently than classical algorithms. This translates to significant speed advantages, accelerating the drug discovery process.
Case Studies and Real-World Applications of D-Wave's Technology
While specific details of many D-Wave collaborations remain confidential due to the competitive nature of drug discovery, the company has publicly highlighted its involvement in several projects focused on improving various aspects of drug design. These projects often involve collaborating with pharmaceutical companies and research institutions. For example, partnerships have focused on utilizing quantum annealing for:
- Optimizing drug delivery systems: Improving the efficiency and targeting of drug delivery mechanisms to enhance therapeutic outcomes.
- Accelerating molecular simulations: Reducing the time required to model complex molecular interactions and predict drug efficacy.
These collaborations showcase the practical application of D-Wave's quantum computers in tackling real-world drug discovery challenges. While quantifiable results from individual projects may not be publicly available due to intellectual property considerations, the progress made demonstrates the technology's potential.
The Role of Hybrid Quantum-Classical Algorithms
D-Wave's approach isn't solely reliant on quantum computing. Instead, it employs a hybrid quantum-classical strategy. This approach combines the strengths of both classical and quantum computing:
- Classical computing: excels at data preprocessing, algorithm control, and post-processing of quantum results.
- Quantum annealing: offers a speed advantage in solving specific optimization problems inherent in drug design, such as finding the optimal configuration of molecules.
This hybrid approach maximizes the effectiveness of both technologies, making quantum computing a practical tool for drug discovery, even with current hardware limitations.
The Future Potential of Quantum AI in Drug Discovery
The applications of quantum AI in drug discovery extend far beyond current capabilities. The future holds even more transformative possibilities:
Accelerating Drug Development Through Quantum Machine Learning
Quantum machine learning (QML) algorithms hold enormous potential for analyzing massive datasets of molecular information. This includes:
- Identifying potential drug candidates: QML can identify patterns and correlations that are undetectable by classical algorithms, potentially leading to the discovery of novel drug candidates.
- Predicting efficacy and safety: QML can predict the efficacy and safety of drug candidates with greater accuracy, reducing the need for extensive and costly clinical trials.
Algorithms like Variational Quantum Eigensolver (VQE) and Quantum Approximate Optimization Algorithm (QAOA) are being explored for these applications, promising to significantly shorten the drug development timeline.
Improving Drug Target Identification and Validation
Identifying and validating promising drug targets is a crucial, yet often lengthy and expensive, step in drug discovery. Quantum computing can revolutionize this process:
- Quantum simulations: Accurately simulating the interactions between drug candidates and their target proteins, improving the prediction of drug efficacy and reducing the risk of failure in later stages of development.
- Quantum optimization: Optimizing the design of drug candidates to maximize their binding affinity and efficacy, leading to more effective drugs.
Personalized Medicine and Quantum AI
The ultimate goal of many pharmaceutical advancements is personalized medicine. Quantum AI can play a pivotal role in achieving this by:
- Tailoring treatments: Using individual patient genetic data to predict drug responses and personalize treatment plans.
- Reducing adverse effects: Identifying patients who are more likely to experience adverse drug reactions, allowing for proactive risk management.
This personalized approach promises more effective and safer treatments for individual patients. However, ethical considerations and data privacy issues will need to be addressed as this field advances.
Challenges and Limitations of Quantum AI in Drug Discovery
Despite its enormous potential, Quantum AI in drug discovery faces challenges:
Hardware Limitations and Scalability
Current quantum computers, including those from D-Wave, still have limitations:
- Qubit coherence: Maintaining the quantum state of qubits for sufficient durations to perform complex computations remains a challenge.
- Error rates: Quantum computations are susceptible to errors, requiring advanced error correction techniques.
- Scalability: Building larger and more powerful quantum computers is crucial for tackling the most complex drug discovery problems.
Data Availability and Quality
Effective QML algorithms require vast amounts of high-quality data:
- Data acquisition: Obtaining comprehensive and reliable data on molecular structures, interactions, and biological responses is often difficult and expensive.
- Data preprocessing: Preparing data for use in quantum algorithms can be time-consuming and require significant expertise.
- Data standardization: Establishing consistent data formats and standards across different research groups is essential for facilitating collaboration and data sharing.
Expertise and Workforce Development
The field requires highly specialized expertise:
- Interdisciplinary collaboration: Successful development requires collaboration between quantum computing experts, drug discovery scientists, and bioinformaticians.
- Workforce development: Significant investment in education and training is needed to build a skilled workforce capable of driving innovation in this field.
Conclusion
D-Wave (QBTS) is making significant strides in applying quantum annealing to address computationally challenging aspects of drug discovery. The potential of Quantum AI in drug discovery to accelerate development, improve accuracy, and enable personalized medicine is undeniable. While challenges remain in hardware limitations, data availability, and workforce development, the ongoing advancements are paving the way for a future where life-saving medications are developed faster and more effectively. Explore the potential of Quantum AI in Drug Discovery with D-Wave (QBTS) and learn how this revolutionary technology is changing the future of medicine.
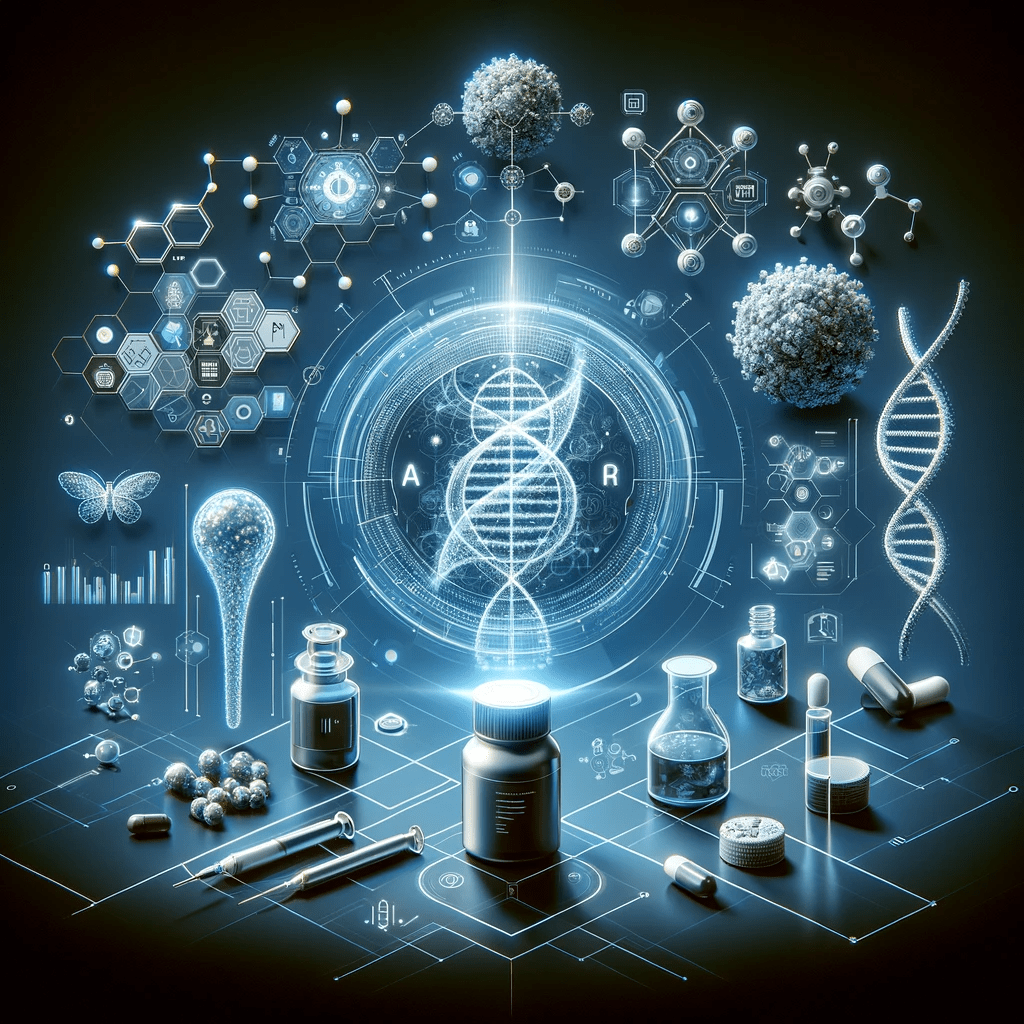
Featured Posts
-
Biarritz Hommage Aux Femmes Programme Du 8 Mars
May 20, 2025 -
Hamilton Ferrari Clash Tea Break Tensions At Miami Gp
May 20, 2025 -
Agatha Christies Poirot The Enduring Appeal Of The Master Detective
May 20, 2025 -
Abc News Show Cancellations Possible After Extensive Layoffs
May 20, 2025 -
Will Increased Control Stifle Londons Live Music Festivals
May 20, 2025
Latest Posts
-
Suomi Mm Karsinnoissa Huuhkajien Valmennuksen Uudistuminen
May 20, 2025 -
Wwe Raw Tyler Bate Returns Forming Tag Team With Pete Dunne
May 20, 2025 -
The Future Of Aj Styles In Wwe Contract Talks And Speculation
May 20, 2025 -
Huuhkajien Uusi Valmennus Ja Mm Karsinnat
May 20, 2025 -
Aj Styles Contract Situation A Wwe Update
May 20, 2025