Surveillance Concerns In AI-Based Mental Health Treatment
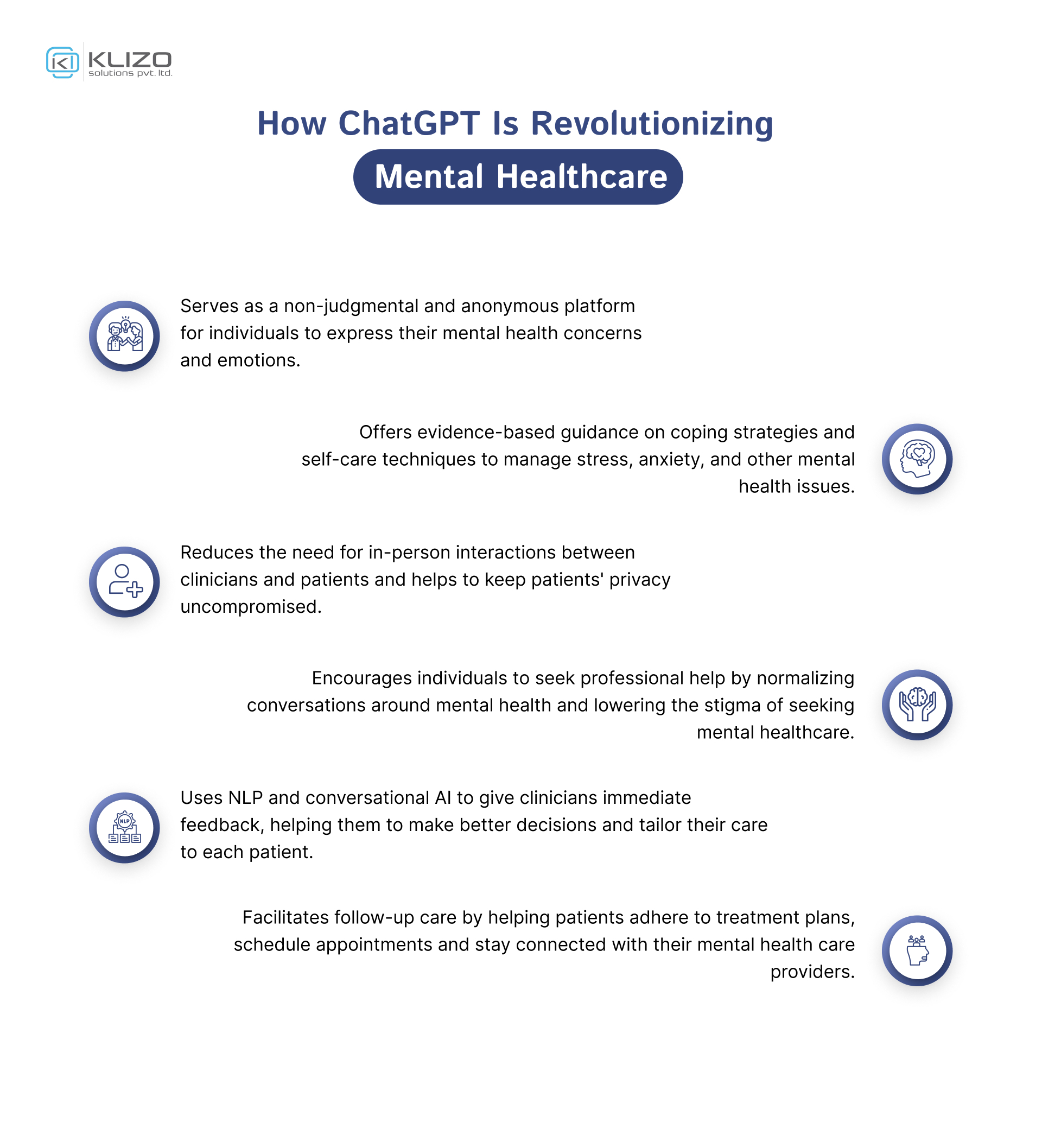
Table of Contents
Data Privacy and Security Risks in AI Mental Healthcare
Mental health data is inherently sensitive, revealing deeply personal information about an individual's thoughts, feelings, and behaviors. The potential consequences of a data breach in this context are severe. AI systems, despite their sophistication, are vulnerable to hacking and data theft, jeopardizing the confidentiality of patient information. This vulnerability is magnified by the often-complex interconnectedness of AI systems and their reliance on cloud-based storage and processing.
- Unauthorized access to patient records: A successful cyberattack could expose sensitive mental health diagnoses, treatment plans, and personal details to unauthorized individuals or organizations.
- Data breaches leading to identity theft and discrimination: Stolen data could be used for identity theft, blackmail, or discriminatory practices, potentially causing significant harm to individuals already facing vulnerability.
- Lack of robust data encryption and anonymization techniques: Inadequate security measures, such as insufficient encryption or incomplete data anonymization, leave patient data susceptible to exploitation.
- The potential for data misuse by third-party vendors: The involvement of multiple vendors in the development and deployment of AI systems increases the risk of data breaches and misuse.
Compliance with regulations like HIPAA (Health Insurance Portability and Accountability Act) in the US and GDPR (General Data Protection Regulation) in Europe is crucial, but ensuring complete data privacy and security in the context of AI remains a significant challenge.
Algorithmic Bias and Discrimination in AI Mental Health Tools
AI algorithms are trained on data, and if this data reflects existing societal biases, the resulting AI system will likely perpetuate and even amplify those biases. This is particularly problematic in mental healthcare, where biased algorithms could lead to inaccurate diagnoses, inappropriate treatment plans, and discriminatory outcomes for certain demographic groups.
- Bias in training data leading to skewed outcomes: If the data used to train an AI algorithm underrepresents certain populations or overrepresents others, the algorithm will likely produce biased results.
- Lack of diversity in AI development teams: A lack of diversity among AI developers can lead to a lack of awareness of potential biases embedded in algorithms.
- The potential for AI to perpetuate existing societal biases: AI systems can inadvertently reinforce existing societal inequalities related to race, gender, socioeconomic status, and other factors.
- The need for algorithmic transparency and accountability: Understanding how an AI algorithm arrives at its conclusions is essential to identify and correct biases. Accountability mechanisms are needed to hold developers responsible for addressing algorithmic bias.
Lack of Transparency and Explainability in AI-Driven Mental Healthcare
Many AI algorithms used in mental healthcare operate as "black boxes," meaning their decision-making processes are opaque and difficult to understand. This lack of transparency raises significant concerns about patient trust, informed consent, and the ability to identify and correct errors.
- Difficulty in identifying and correcting errors in AI systems: Without understanding how an AI system arrived at a particular conclusion, it is difficult to identify and correct errors or biases.
- Lack of clear guidelines on how AI decisions are made: Patients and clinicians need clear explanations of how AI systems make decisions to ensure informed consent and trust.
- Challenges in ensuring patient understanding of AI-driven interventions: Complex AI systems can be difficult for patients to understand, hindering meaningful engagement with treatment.
- The need for explainable AI (XAI) in mental healthcare: Developing explainable AI systems that provide clear and understandable explanations of their decisions is essential for building trust and ensuring ethical use.
The Impact of Constant Monitoring on Patient Autonomy and Mental Well-being
AI-powered monitoring tools offer the potential for continuous assessment of mental health, but this constant surveillance raises serious concerns about patient autonomy and mental well-being. The potential for increased anxiety, stress, and feelings of being controlled could negatively impact a patient's recovery.
- Increased feelings of anxiety and stress due to constant monitoring: The feeling of being constantly watched can exacerbate anxiety and stress, hindering recovery.
- Erosion of trust between patients and clinicians: Over-reliance on AI monitoring could lead to a diminished therapeutic relationship and erode trust between patients and clinicians.
- The potential for AI to discourage open communication and self-disclosure: Fear of judgment or negative consequences might discourage patients from openly sharing their thoughts and feelings.
- The importance of balancing monitoring with patient autonomy: It's critical to strike a balance between effective monitoring and respecting patient autonomy and privacy.
Mitigating Surveillance Concerns in AI-Based Mental Health Treatment
Addressing the surveillance concerns associated with AI in mental healthcare requires a multi-faceted approach focusing on robust security measures, algorithmic fairness, transparency, and ethical guidelines.
- Implementing robust data security measures: Strong encryption, secure data storage, and regular security audits are essential to protect patient data.
- Developing bias-mitigation techniques for AI algorithms: Techniques like data augmentation and algorithmic fairness checks can help mitigate biases in AI systems.
- Promoting transparency and explainability in AI systems: Developing explainable AI (XAI) techniques will enable clinicians and patients to understand how AI systems arrive at their conclusions.
- Establishing clear ethical guidelines and regulations: Clear ethical guidelines and robust regulations are needed to govern the development and deployment of AI in mental healthcare.
- Prioritizing patient consent and control over data: Patients must have clear control over their data and be fully informed about how it is being used.
Conclusion
The integration of AI in mental healthcare offers significant potential benefits, but it also presents substantial surveillance concerns in AI-based mental health treatment. Addressing data privacy and security risks, algorithmic bias, lack of transparency, and the impact of constant monitoring is paramount to ensure ethical and responsible innovation in this field. We must prioritize patient rights, data protection, and algorithmic fairness to harness the transformative power of AI while mitigating its potential harms. We encourage readers to actively engage in further discussion and research on these crucial issues to foster responsible innovation and advocate for ethical AI development and deployment in mental healthcare.
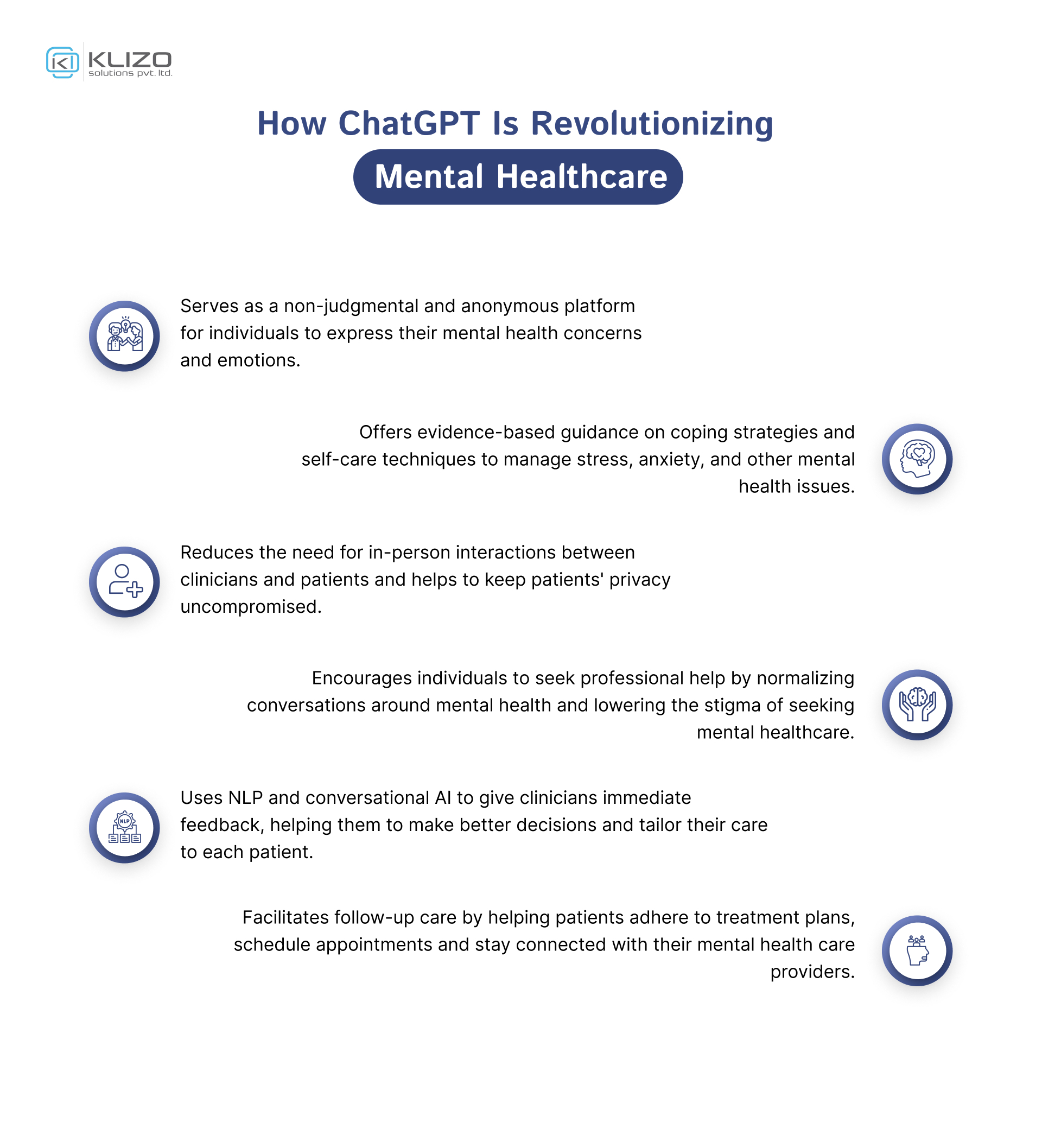
Featured Posts
-
Thoi Gian Xong Hoi Phu Hop Voi Tung Doi Tuong
May 16, 2025 -
Paddy Pimbletts Ufc 314 Travel The Influence Of Liverpool Football Club
May 16, 2025 -
Finding The Right Black Decker Steam Iron Ultimate Guide
May 16, 2025 -
Rethinking Middle Management Their Essential Contribution To Business Growth
May 16, 2025 -
Athletic Club De Bilbao News Analysis And More From Vavel Usa
May 16, 2025
Latest Posts
-
Former All Star Jake Peavy Named Special Assistant To Padres Ceo
May 16, 2025 -
Ilia Topuria Vs Paddy Pimblett The Ufc Showdown We Need
May 16, 2025 -
Ufc 314 Pimbletts Next Fight Ilia Topuria
May 16, 2025 -
Paddy Pimbletts Ufc 314 Hit List Ilia Topuria Leads The Charge
May 16, 2025 -
Padres Roster Update Merrills Return Campusanos Demotion
May 16, 2025