The Impact Of Outdated Business Applications On AI Adoption
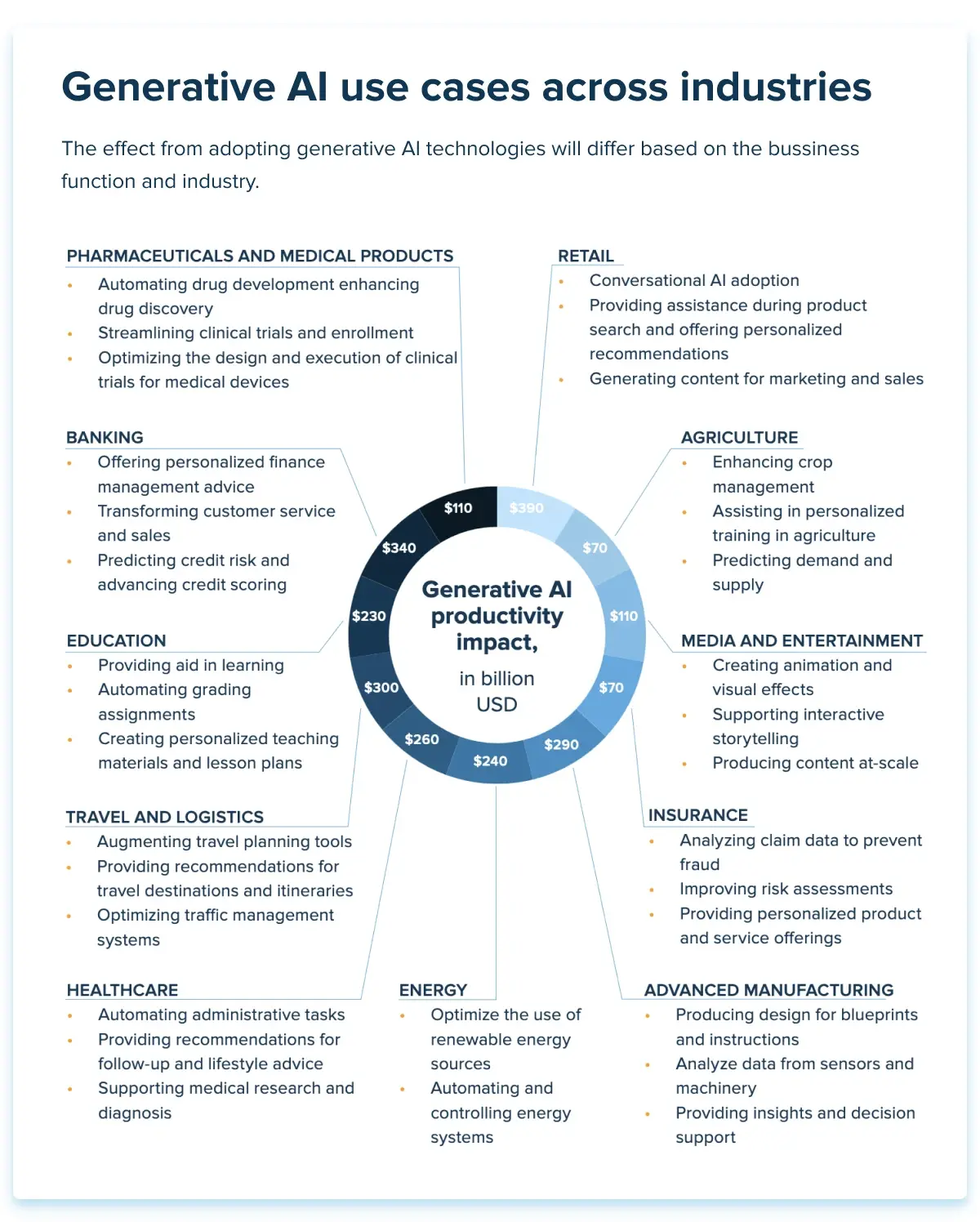
Table of Contents
Data Silos and Integration Challenges
Outdated systems frequently present significant data integration hurdles, directly impacting AI adoption. The problem stems from a lack of cohesive data infrastructure, leading to challenges in accessing and processing the comprehensive datasets AI algorithms require for effective analysis.
Data Incompatibility
Outdated systems often store data in disparate formats and locations, creating data silos that are difficult to reconcile. This incompatibility inhibits AI's ability to create a holistic view of business operations.
- Lack of standardized data formats: Different departments might use different databases and file formats, creating incompatibility issues.
- Difficulty in data extraction and transformation: Extracting data from disparate systems requires significant manual effort and specialized tools, increasing the risk of errors.
- Increased risk of data errors: Manual data transfer is prone to human error, leading to inconsistencies and inaccuracies in the data used for AI model training.
- Inability to leverage data for holistic insights: Without a unified view of data, AI algorithms cannot deliver comprehensive and insightful analyses crucial for informed decision-making.
Limited API Access
Legacy systems often lack robust Application Programming Interfaces (APIs), hindering seamless integration with modern AI platforms and tools. This limitation necessitates inefficient and error-prone manual data transfer processes.
- Manual data transfer processes: The absence of APIs forces manual data transfer, a time-consuming and error-prone task.
- Increased risk of human error: Manual processes increase the likelihood of data entry errors, affecting the accuracy and reliability of AI models.
- Bottlenecks in data flow: Manual data transfer creates bottlenecks, slowing down AI model training and deployment.
- Slower AI model training and deployment: The delays caused by manual data handling significantly hinder the speed and efficiency of the entire AI implementation process.
Scalability and Performance Issues
The limitations of outdated applications extend to scalability and performance, significantly impacting the effectiveness of AI initiatives. These legacy systems are often ill-equipped to handle the computational demands and data volumes inherent in AI processes.
Infrastructure Limitations
Outdated applications are often built on outdated infrastructure that struggles to meet the computational demands of sophisticated AI algorithms. This often results in performance bottlenecks and system instability.
- Slow processing speeds: Legacy systems might not have the processing power needed for complex AI algorithms, leading to slow response times and reduced efficiency.
- System crashes and downtime: The inability to handle the increased load from AI processes can result in frequent system crashes and downtime.
- Inability to handle large datasets: Legacy systems are often limited in their capacity to store and process large datasets essential for effective AI model training.
- Limited scalability to accommodate future growth: As AI initiatives expand, outdated systems may struggle to scale, leading to performance issues and limitations in AI deployment.
Maintenance and Support Costs
Maintaining and supporting legacy systems can be extremely expensive, diverting resources away from crucial AI investments. Finding skilled personnel to maintain these outdated systems also presents a significant challenge.
- High maintenance expenses: The cost of maintaining and patching outdated systems can be substantial.
- Difficulty in finding skilled personnel: Finding individuals with the expertise to maintain legacy systems is increasingly difficult as these technologies become obsolete.
- Security vulnerabilities: Outdated systems often have unpatched security vulnerabilities, increasing the risk of data breaches and security incidents.
- Lack of vendor support: Many vendors no longer support legacy systems, leaving organizations vulnerable to security risks and lacking access to timely updates.
Security Risks and Data Governance
Outdated business applications introduce significant security vulnerabilities and data governance challenges, posing serious risks to AI projects and compliance.
Security Vulnerabilities
Legacy systems are often riddled with security vulnerabilities that expose sensitive data to cyber threats, compromising AI projects and potentially leading to severe legal and financial repercussions.
- Outdated security protocols: Many legacy systems lack the updated security protocols necessary to protect against modern cyber threats.
- Increased risk of data breaches: Vulnerabilities in legacy systems increase the likelihood of data breaches, leading to significant financial and reputational damage.
- Non-compliance with data privacy regulations: Legacy systems may not meet current data privacy regulations, leading to potential legal penalties.
- Reputational damage: Data breaches and non-compliance can severely damage an organization's reputation and erode customer trust.
Data Governance Challenges
Older systems often lack robust data governance capabilities, making it difficult to ensure data quality, integrity, and compliance with regulatory requirements.
- Lack of data quality controls: Legacy systems often lack built-in data quality controls, leading to inconsistencies and inaccuracies.
- Difficulty in tracking data lineage: Tracing the origin and movement of data is crucial for data governance but is challenging with older systems.
- Non-compliance with regulations: Failure to meet data governance standards can result in legal penalties and fines.
- Increased risk of legal penalties: Non-compliance with data privacy and security regulations can result in substantial fines and legal repercussions.
Strategies for Overcoming Challenges
Successfully integrating AI requires addressing the limitations imposed by outdated business applications. This necessitates a strategic approach focusing on modernization, improved data integration, and a shift to more robust infrastructure.
Cloud Migration
Migrating to the cloud offers a pathway to overcome many challenges related to outdated business applications. Cloud platforms provide the scalability, flexibility, and advanced data management capabilities required to support sophisticated AI initiatives.
- Increased scalability and availability: Cloud platforms offer virtually limitless scalability to handle growing data volumes and computational demands.
- Cost optimization: Cloud-based solutions can reduce IT infrastructure costs associated with maintaining legacy systems.
- Improved data security: Cloud providers invest heavily in security, often offering stronger security than many on-premise legacy systems.
- Enhanced data integration capabilities: Cloud platforms offer tools and services that simplify data integration across disparate systems.
Software Modernization
Software modernization involves refactoring or replacing legacy applications with modern, cloud-native solutions designed for seamless AI integration. This approach addresses many of the performance, security, and scalability issues associated with legacy systems.
- Improved system performance: Modern applications offer superior performance and efficiency compared to their legacy counterparts.
- Enhanced security: Modern applications incorporate updated security features and protocols, minimizing vulnerabilities.
- Increased agility and flexibility: Modern applications are more adaptable to changing business needs and can be more easily integrated with AI tools.
- Reduced maintenance costs: Modern applications often require less maintenance and support, reducing operational costs.
Data Integration Strategies
Implementing robust data integration platforms is vital for connecting disparate data sources and improving data quality, crucial factors for effective AI model training and deployment.
- Improved data visibility: Data integration platforms provide a unified view of data from various sources, improving data transparency.
- Enhanced data quality: Data integration processes can cleanse and transform data, enhancing its accuracy and reliability.
- Easier data access: Data integration platforms simplify data access for AI algorithms, speeding up model development and deployment.
- Faster AI model development: Improved data accessibility and quality accelerate the AI model development process.
Conclusion
Outdated business applications pose significant impediments to successful AI adoption. Data silos, scalability issues, security risks, and high maintenance costs associated with legacy systems can severely hinder AI implementation and limit its potential benefits. By addressing these challenges through strategic cloud migration, software modernization, and the implementation of robust data integration strategies, organizations can pave the way for seamless AI adoption and unlock its transformative power. Don't let outdated business applications impede your AI journey – assess your current systems and implement a plan for modernization to fully leverage the potential of AI. Embrace digital transformation and modernize your business applications to unlock the true power of AI.
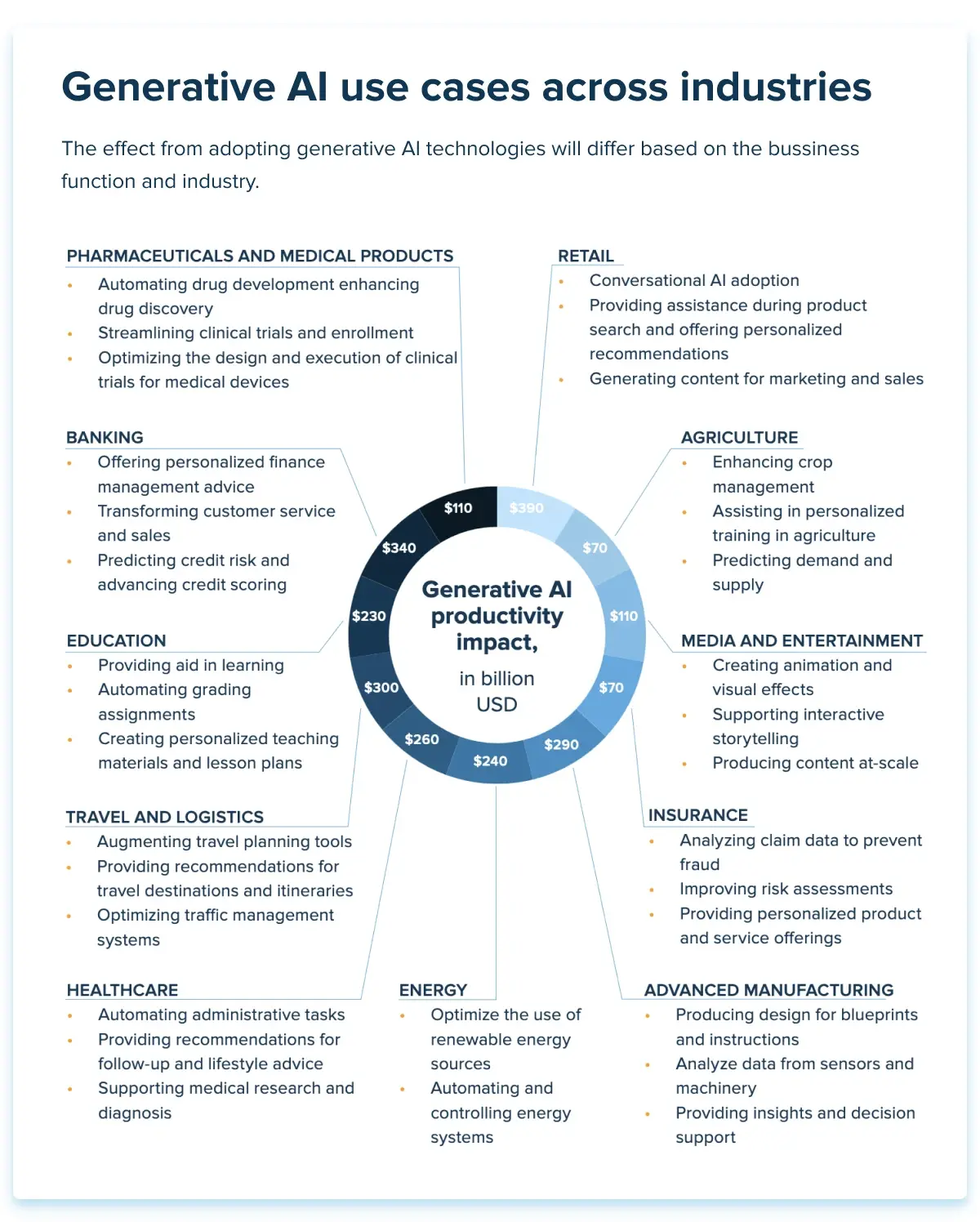
Featured Posts
-
Internal Spd Vote Looms Campaigning For Coalition Agreement In Germany
Apr 30, 2025 -
Jury Selection Process Starts In Fatal Charlotte Case
Apr 30, 2025 -
Naacp Image Awards 2024 Beyonce Blue Ivy And Kendrick Lamars Big Night
Apr 30, 2025 -
Police Accountability Review Campaigners Raise Serious Concerns
Apr 30, 2025 -
Disney Layoffs 200 Jobs Cut Across News And Entertainment
Apr 30, 2025
Latest Posts
-
Analyzing The Panthers Strategy With Their 8th Draft Pick
May 01, 2025 -
Michael Jordan Fast Facts And Key Stats
May 01, 2025 -
Will The Panthers 8th Pick Deliver Another Star
May 01, 2025 -
Coronation Street Daisy Midgeleys Exit Story Revealed
May 01, 2025 -
Farmers And Foragers Charlottes Old Lantern Barn Available For Purchase
May 01, 2025