Turning "Poop" Into Podcast Gold: An AI-Powered Approach To Repetitive Document Analysis
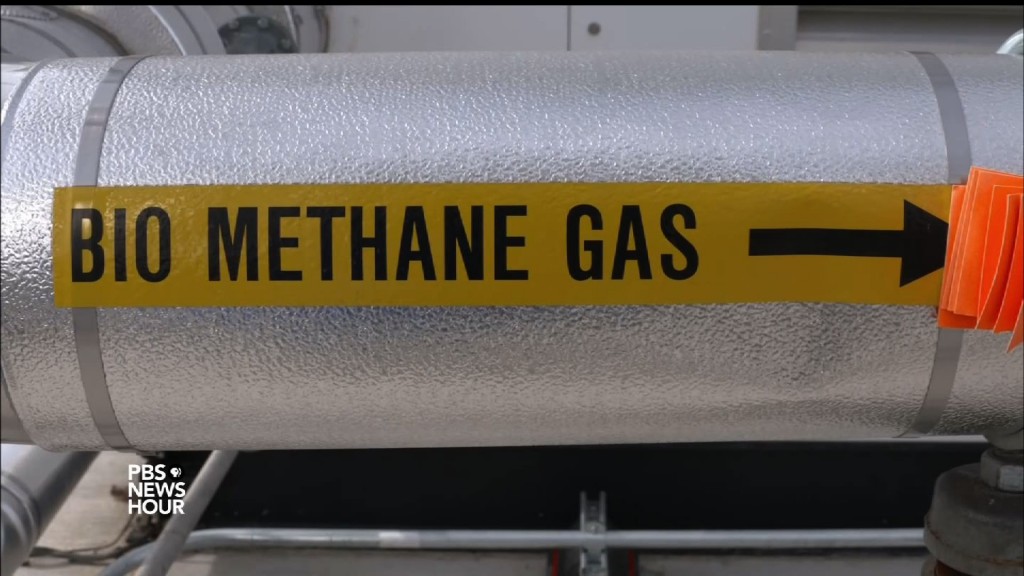
Table of Contents
Identifying the Problem: The High Cost of Manual Repetitive Document Analysis
Manual repetitive document analysis is a significant bottleneck for many organizations. The sheer volume of documents, coupled with the need for meticulous accuracy, creates a substantial burden on time, resources, and ultimately, the bottom line.
Time Consumption:
Manual review consumes a massive amount of time, leading to delays, missed deadlines, and hampered productivity. This is particularly true in industries dealing with high document volumes:
- Legal: Reviewing contracts, discovery documents, and legal precedents demands countless hours of meticulous work.
- Medical: Processing patient records, insurance claims, and medical reports requires careful attention to detail, easily leading to bottlenecks.
- Finance: Analyzing financial statements, transaction records, and audit trails is time-consuming and prone to human error.
The impact extends beyond mere delays. The repetitive nature of the work leads to:
- Decreased employee morale: Monotonous tasks can lead to burnout and decreased job satisfaction.
- Increased risk of human error: Fatigue and boredom increase the likelihood of mistakes, potentially leading to costly consequences. A single missed detail in a legal document or medical report can have severe repercussions.
Cost Inefficiency:
The financial burden of manual repetitive document analysis is substantial. Employing human resources for these tasks is expensive, and the potential for errors adds to the overall cost.
- Direct Costs: Salaries, benefits, and training costs associated with employing personnel dedicated to manual document review quickly accumulate. Let's consider a scenario where processing a single document manually takes an hour at a cost of $50/hour (including overhead). Processing 1000 documents would cost $50,000.
- Indirect Costs: The opportunity cost is equally significant. Employees engaged in repetitive tasks could be contributing to more strategic initiatives. Their time could be better spent on tasks requiring higher-level skills and judgment. The cost of lost opportunities due to slow processing is often overlooked but can be substantial.
- Error Costs: Human error can lead to costly legal battles, medical mishaps, or financial inaccuracies, further escalating the overall expense.
AI-Powered Solutions for Efficient Repetitive Document Analysis
Fortunately, AI offers powerful solutions for streamlining repetitive document analysis. By leveraging advanced technologies, organizations can significantly improve efficiency and accuracy.
Natural Language Processing (NLP):
NLP is crucial for extracting meaningful information from unstructured text data. This involves:
- Named Entity Recognition (NER): Identifying and classifying named entities such as people, organizations, locations, dates, and quantities within text.
- Sentiment Analysis: Determining the emotional tone expressed in a document (positive, negative, neutral).
- Topic Modeling: Identifying the main themes and topics discussed in a document.
These techniques automate the identification of key information, significantly reducing the time and effort required for manual review. For example, in legal document review, NLP can automatically extract key clauses, dates, and parties involved.
Machine Learning (ML) for Pattern Recognition:
ML algorithms excel at identifying patterns and anomalies within large datasets. This is particularly valuable for:
- Improved Accuracy: ML models can identify subtle patterns and inconsistencies that might be missed by human reviewers.
- Faster Processing Times: AI can process vast quantities of data much faster than humans, leading to significant time savings.
Both supervised and unsupervised learning methods are applicable. Supervised learning involves training the model on labeled data, while unsupervised learning allows the model to identify patterns without prior labeling.
Optical Character Recognition (OCR):
OCR plays a vital role in converting scanned documents into machine-readable text. This is essential for enabling NLP and ML to process documents in various formats:
- Integration with NLP and ML: OCR acts as the bridge, converting images into text that AI can then analyze.
- Handling Diverse Formats: OCR can handle various document types, including PDFs, images, and handwritten text (though accuracy varies). The importance of accurate OCR cannot be overstated, as errors at this stage will propagate through the entire analysis pipeline.
Implementing AI for Repetitive Document Analysis: A Step-by-Step Guide
Successfully implementing AI for repetitive document analysis requires careful planning and execution.
Data Preparation:
Before deploying AI models, it's crucial to prepare the data for optimal performance. This involves:
- Data Cleansing: Removing irrelevant information, correcting inconsistencies, and handling missing data.
- Format Standardization: Converting documents into a consistent format to ensure seamless processing by AI models.
- Addressing Challenges: Dealing with noisy data, inconsistencies in formatting, and various document types requires careful consideration.
Careful data preparation is paramount for accurate and reliable results.
Model Selection and Training:
Choosing the right AI model and training it effectively are critical steps.
- Model Selection: Different AI models are suited for different tasks. Transformers and recurrent neural networks (RNNs) are commonly used for natural language processing in document analysis.
- Model Training: Training involves feeding the chosen model with a representative dataset to learn the patterns and relationships within the data.
The selection and training of the model directly impact the accuracy and efficiency of the analysis.
Integration and Deployment:
Finally, integrating the AI solution into existing workflows and deploying it for practical use is essential.
- User-Friendly Interfaces: The AI system should have intuitive interfaces to facilitate easy use by non-technical personnel.
- Seamless Integration: Integration with existing systems (e.g., document management systems, CRM) is critical for efficient workflow.
Successful deployment requires careful planning and attention to user experience.
Conclusion:
Turning "poop" work into podcast-worthy productivity is achievable with AI-powered repetitive document analysis. By leveraging NLP, ML, and OCR, businesses can drastically reduce costs, improve accuracy, and free up valuable human resources for more strategic tasks. Don't let tedious document analysis bog you down – explore the power of AI to streamline your processes and unlock significant efficiency gains. Start exploring AI solutions for repetitive document analysis today!
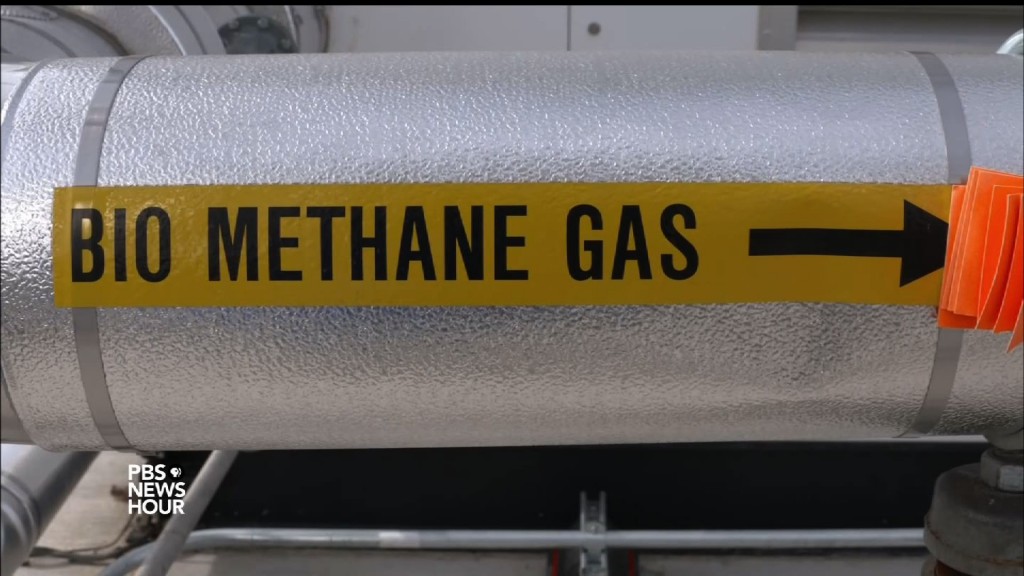
Featured Posts
-
M56 Delays Cheshire Deeside Border Collision Causes Traffic Disruption
May 24, 2025 -
Horoscopo De La Semana 1 Al 7 De Abril De 2025 Todos Los Signos
May 24, 2025 -
Dazi Stati Uniti Prezzi Moda E Tendenze 2024
May 24, 2025 -
Kerings Financial Report Sales Down Guccis New Era Begins
May 24, 2025 -
Glastonbury 2025 Lineup Is It The Strongest Yet Featuring Charli Xcx And Neil Young
May 24, 2025
Latest Posts
-
Sylvester Stallones Tulsa King Season 3 Neal Mc Donoughs Return And Filming Updates
May 24, 2025 -
Is It Going To Rain In Nyc During Memorial Day Weekend
May 24, 2025 -
Find The Best Memorial Day 2025 Sales A Comprehensive Guide
May 24, 2025 -
Memorial Day Weekend Predicting Rain In New York City
May 24, 2025 -
Box Office Prediction Stitchpossibles Path To A Record Breaking 2025 Face Off
May 24, 2025